AI projects in Europe
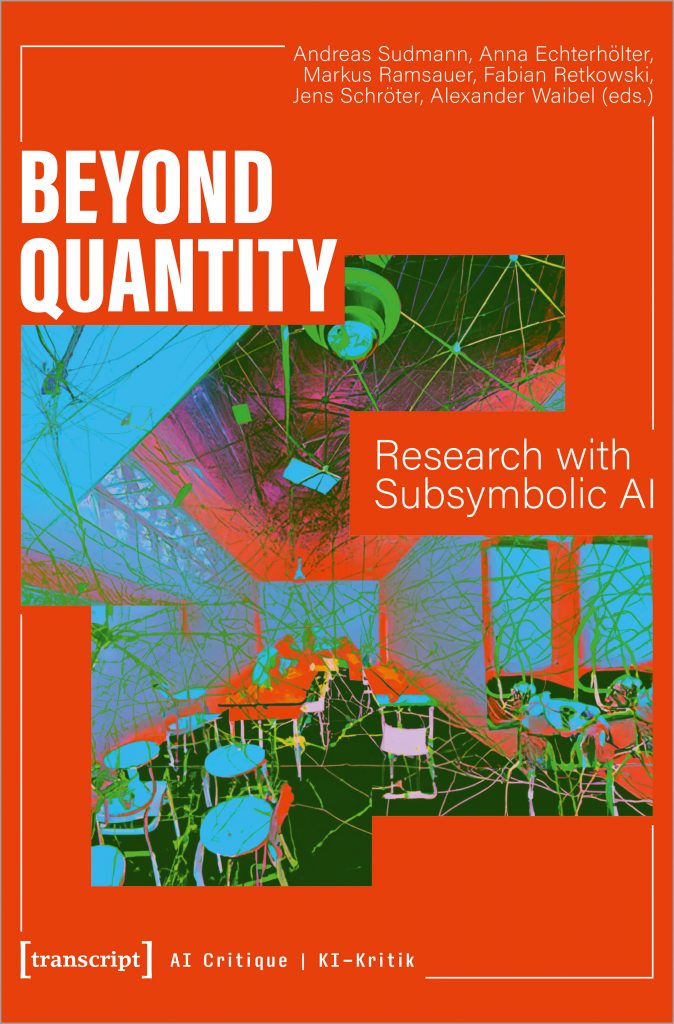
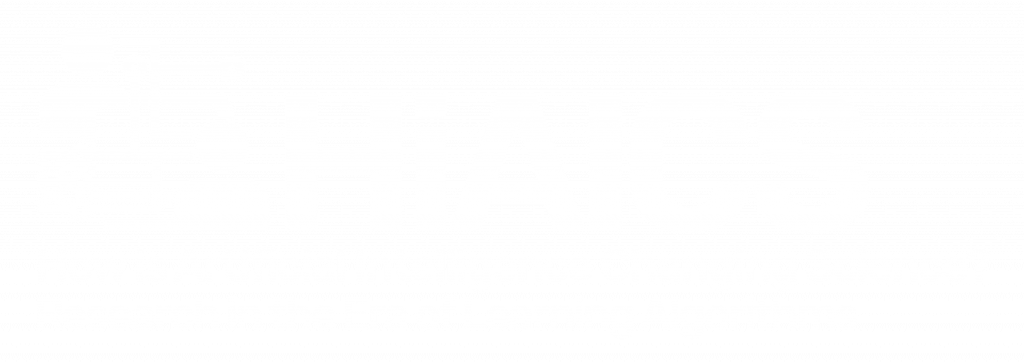
AI Projects in Europe
Selected Disciplines
Below you will find a list of projects at European universities that use different methods of artificial intelligence for their research questions.
As a starting point, we document projects in the fields of medicine, environmental science, sociology, economics, film studies, as well as literary studies and linguistics.
The list of projects and disciplines will be updated and extended continuously.
Medicine
Advanced Robotic Breast Examination Intelligent System (ARTEMIS)
ARTEMIS aims at developing an intelligent robotic system that will help early breast cancer detection. We will develop intelligent algorithms and soft robotic system for this project.
Contact: Amir Ghalamzan
—
AI Clinician: Reinforcement Learning in Intensive Care
Sepsis is the third leading cause of death worldwide and the main cause of mortality in hospitals but the best treatment strategy remains uncertain. In particular, evidence suggests that current practices in the administration of intravenous fluids and vasopressors are suboptimal and likely induce harm in a proportion of patients. To tackle this sequential decision-making problem, we developed a reinforcement learning agent, the Artificial Intelligence (AI) Clinician, which extracted implicit knowledge from an amount of patient data that exceeds by many-fold the life-time experience of human clinicians and learned optimal treatment by analyzing a myriad of (mostly suboptimal) treatment decisions.
Contact: Anthony C. Gordon, A. Aldo Faisal & Matthieu Komorowski
—
AI-Enabled Imaging
Areas of research include: AI for image acquisition, reconstruction and analysis;AI-enabled decision support for diagnosis and prognosis; Novel methodologies that address the challenges in translating AI solutions into the clinic.
Contact: Bernhard Kainz & Emma Robinson
—
AiBle Project
AiBle is a UK/France cross-border EU Interreg project to improve the recovery experience of stroke patients with better treatment effects and efficiency by developing an upper-limb rehabilitation exoskeleton robot based on AI and cloud computing.
This project aims to develop a new generation of exoskeleton robot that will benefit stroke patients by providing advanced functionality that will enable remote but active rehabilitation. This will be achieved by the integration of artificial intelligence, virtual reality and cloud computing.
Contact: Venky Dubey
—
AIcope – AI support for Clinical Oncology and Patient Empowerment
While there are various efficacious treatments available for most cancers, many of them may drastically impact the entire patients’ life, each in their own way. To further reduce the societal burden of cancer, it is therefore crucial to pick the right type of treatment, not only based on the patient’s biological profile but also on their preferences and general lifestyle.
Unfortunately, this is seldom feasible. One of the main reasons are the severe time constraints of contemporary clinical practice—it is virtually impossible to review the implications of various available treatments with the patients while truly taking both their biomedical and their lifestyle profiles into account. And this is the very real, pressing clinical need that motivates the AIcope project. To address the need, we will: (i) Collect, extract and preprocess data from oncological patient records and relevant public datasets on diseases, interventions and drugs. (ii) Integrate the preprocessed data in a uniform, semantically-interlinked resource (a knowledge graph) and augment it by inferred links. (iii) Develop question answering and visual exploration interfaces on top of the knowledge graph in a co-design process with doctors, patients and clinical psychologists. (iv)Evaluate the resulting decision support prototype by its preliminary deployment in clinical settings and comparison with the current practices in the treatment selection process.
Contact: Vít Nováček
—
AIR Lund – Artificially Intelligent use of Registers
The demographic transition of society and new technology contribute to exponential accumulation of micro-data in healthcare registers. Novel usage of artificially intelligent decision aids, applied on the Swedish register infrastructure, holds promises for improved quality and efficiency of healthcare. Focussing on cardiometabolic diseases, AIR Lund will critically assess the added value of machine learning compared to standard statistical approaches for predictions and decision aids in three specific settings: 1) prevention, where we hope to identify new groups of hidden high-risk individuals and new sets of modifiable risk factors; 2) diagnosis, where we in emergency care hope to improve general risk assessment and diagnosis of acute coronary disease; 3) prognosis, where we hope to improve long-term predictions and identify new risk patterns that forego adverse patient outcomes and high healthcare needs.
Contact: Jonas Björk, Mattias Ohlsson, Olle Melander et al.
—
Analysis and development of an electronic frailty index (eFI) for Finnish healthcare to identify at-risk individuals at early stages
The degree of frailty is a critical determinant of healthy aging and a predictor of various adverse outcomes, such as mortality, disability and high healthcare utilization. Frailty is nevertheless reversible if identified at an early stage, but methods to assess frailty in routine healthcare practice are limited and middle-aged and younger adults are completely overlooked.
This project aims to analyze and create an electronic frailty index (eFI), a tool for Finnish healthcare to facilitate early identification of vulnerable at-risk individuals. The eFI can also facilitate planning proactive care pathways, guide decision-making and preoperative screening. The eFI allows tracking of health and functioning not only at an individual level but also in populations across different geographical and social areas. Artificial intelligence will be an integral part of the analytical work. We will devise machine learning algorithms to select the eFI items exhibiting the highest predictive accuracy and generalizability, andidentify eFI items in unstructured data, i.e., free-text clinical notes.
Contact: Juulia Jylhävä
—
ARAMIS Lab
Contact: Olivier Colliot & Stanley Durrleman
—
Auswirkungen von EDV im intensivmedizinischen Umfeld
Insbesondere die Untersuchung von Nutzerzufriedenheit, Veränderungen von Arbeitsabläufen, Auswirkungen auf verschiedene Berufsgruppen sowie Kosten-Nutzen-Analysen und Auswirkungen auf die Behandlungsqualität und das Behandlungsergebnis stehen hier im Fokus. Die Daten der elektronischen Patientenakte werden systematisch auf neue und unbekannte Sachverhalte untersucht. Dazu werden neue Methoden zur Krankheitsfrüherkennung, komplexen Alarmierung und klinischen Entscheidungsunterstützung entwickelt. Das Ziel dabei ist, die Behandlungsqualität und die Patientensicherheit zu verbessern und so einen zusätzlichen klinischen Nutzen aus dem EDV-Einsatz im intensivmedizinischen Umfeld zu generieren.
Contact: Ixchel Castellanos
—
Automatic Question Generation for Occupational Health Assessment
In this project, UH will help Heales Enterprises Ltd (HEL) to set up an automatic question generation system using advanced Machine Learning methods. This system will be used in Occupational Health Assessment.
Contact: Yi Sun & Farshid Amirabdollahian
—
Barsnes Group
The main objective of the Barsnes Group is to combine state-of-the-art bioinformatics research with the current biomedical knowledge, thus building a bridge between project specific high-throughput omics analyses and novel biomedical knowledge.
Contact: Harald Barsnes
—
Biomedical Image Analysis
Rapid advances in non-invasive neuroimaging methods have revolutionized the possibilities to study changes occurring in living brain across a variety of time-scales ranging from seconds to an entire life span. A large part of these advances can be attributed to the development of dedicated computational algorithms and applied mathematics, which are essential to extract quantitative information from images. My group develops these computational methods to analyze the brain imaging data, evaluates the methods, for example, by using advanced simulations, and together with collaborators applies these methods to study the brain.
Contact: Jussi Tohka
—
Boosting-RF: Development and added value of boosting with penalized splines and random forests in excess mortality hazard models applied to cancer epidemiology
In cancer epidemiology, net survival and the dynamics of excess hazard according to the time since diagnosis are both major indicators and evaluating the effect of prognosis variables on these indicators are important.
In the current context of development of health data platforms, registry or cohort data may be augmented with various new data sources.
Two complementary techniques originating from machine learning will be extended to net survival setting. First, we will extend Boosting techniques to excess hazard models, using multidimensional penalized splines as base learners. Second, we will develop a random forest methodology for net survival estimation. Then, the performance of these methods will be evaluated through simulation.
These methods would benefit the community by increasing the ability to analyze rich data, with possibly complex phenomena, in a (net) survival setting.
Contact: Roch Giorgi
—
Center for Computational Imaging and Simulation Technologies in Biomedicine (CISTIB)
The Center for Computational Imaging and Simulation Technologies in Biomedicine is an interdisciplinary centre that conducts research in computational medicine, spanning the University of Leeds‘ School of Computing and The Faculty of Medicine and Health.
Our current research can be grouped into various areas: Artificial intelligence in medical imaging; computational imaging phenomics; patient-specific modelling and simulation; in silico clinical trials for medical devices; massive-scale health data sharing, modelling and simulation platforms.
Contact: Alejandro Frangi, Zeike Taylor, Ali Gooya & Toni Lassila
—
Computational Transcriptomics and Evolutionary Bioinformatics Laboratory
The laboratory deals with computer analysis of the whole genome sequencing data obtained in the laboratory itself, by its collaborators, as well as presented in the public domain. The whole genome sequencing is reading and deciphering the sequence of DNA or RNA from any biological sample. The analysis of these data allows us to reveal the genetic programs built into the DNA of organisms, as well as their variation in pathology and environmental changes.
Contact: Elena Zemlyanskaya
—
Dementia Management and Support System
DMSS main purpose is providing support for physicians in their diagnostic reasoning and choice of interventions when meeting new patients with a suspected dementia disease. Managing uncertain information and conflicting medical guidelines using state-of-the art artificial intelligence theories is one line of research, another is developing person-tailored support for reasoning and knowledge development in the medical professional.
Contact: Helena Lindgren
—
Diagnosing Kidney Transplant Diseases
The group developed a pipeline for comprehensive and reproducible analysis of hundreds to thousands of proteins from kidney tissue by SWATH mass spectrometry. With this seed grant, this multidisciplinary team with expertise on pathology, proteomics and AI will determine whether a novel ensemble machine learning feature selection strategy can be used to determine robust proteomics-based patterns that are able to differentiate between different disease states with the final goal to translate these findings into practice and aid with clinical decision making.
Contact: Jesper Kers
—
Diagnostic Biomarkers in Atrial Fibrillation – Autonomic Nervous System Response as a Sign of Disease Progression
Atrial fibrillation (AF) is the most common arrhythmia encountered in clinical practice. To cut costs and reduce patient suffering, tools that help clinicians to optimize personalized AF treatment are needed. Our hypothesis is that autonomic nervous system (ANS) induced modulation in cardiac activity can be used as a diagnostic biomarker of arrhythmia progression. Although AF has received much attention in the scientific community, there is still a complete lack of tools for analysis of ANS modulation in AF. Developing such tools is challenging, since ANS induced modulation in AF results from complex interactions between the ANS, the atria, and the AV node.In this project, we will combine signal processing, cardiac computational modeling and machine learning to develop optimized tools for non-invasive assessment cardiac ANS induced modulation in AF.
Contact: Frida Sandberg, Mikael Wallman & Pyotr Platonov
—
DOLF – Death to Onchocerciasis and Lymphatic Filariasis
To enhance efforts to control and eliminate lymphatic filariasis and onchocerciasis through the optimization of drug therapies and development of strategies for mass drug administration. Our name says it all. Death to Onchocerciasis and Lymphatic Filariasis. This project, supported by the Bill and Melinda Gates Foundation, includes an ambitious set of complementary applied research projects that share the common goal of optimizing therapy to accelerate the elimination of LF and Onchocerciasis. In addition, the project aims to improve chances for LF and/or Oncho control in regions of Africa and Asia that are behind the progress of other countries. We develop strategies to ensure that areas with low acceptance, hard to reach populations, and persistent infection benefit from drug therapy research. We convene with international partners including: DNDi, the World Health Organization, the Ghana University of Health and Allied Sciences, and others to ensure timely dissemination of our results.
Contact: Achim Hoerauf
—
Drug Design, Proteomics and Theorem Proving
Work by Sean Holden applies Bayesian inference, probabilistic programming, and computational learning theory to drug design, proteomics, and theorem proving.
Contact: Sean Holden
—
Exploration of machine learning for pre-emptive scheduling
The project aims to provide and validate new approaches for machine learning in prioritised task scheduled working queues in mega-kernels executed on single instruction multiple data (SIMD) computing units. A working demonstrator using a complex real world algorithm for motion correction in fetal MRI will be used and validated on real, motion corrupted MRI data. With the proposed learning strategies, it is expected to provide accurate reconstructions of the fetal anatomy in-utero and a general framework for the parallelisation of otherwise highly complex computational methods. The fundamental GPU computing methods provide a versatile framework, which will be extended with machine learning methods to automatically and intelligently define task priorities.
Contact: Biomedical Image Analysis Group
—
Exploring the dynamics of biological systems
Led by Dr Bianca Dumitrascu, this group studies how local molecular rules give raise to emergent spatial patterns in the context of biological dynamical systems. Its focus is the use of techniques from statistical optimization, statistical physics and domain adaptation to identify contextual phenotypes in spatial transcriptomic data and to understand the identity of single cells and their interactions in early developement. Projects also explore active learning and graphical neural networks as models to study the effects and side-effects of drug cocktails.
Contact: Bianca Dumitrascu
—
EZMammo
Développement d’un algorithme d’intelligence artificielle pour l’interprétation de mammographies de dépistage.
Contact: Isabelle Thomassin
—
FlowCat – Automated Classification of B-cell Lymphoma Sub Types
In our project, we seek to establish an approach for automated classification of lymphoma subtypes through a deep-learning based predictive model using information from flow cytometry data thereby reducing the need for manual gating.
Contact: Peter Krawitz
—
Forschung am ZMNH Institut für Medizinische Systembiologie
Das primäre Ziel der Forschung am Institut ist es, menschliche Krankheiten besser zu verstehen. Der Fokus liegt dabei auf Erkrankungen des zentralen Nervensystems. Um dieses Ziel zu erreichen, müssen große Mengen heterogener biomedizinischer Daten überprüft und miteinander integriert werden. Wir entwickeln dafür unsere eigenen Systeme, die diese Aufgaben soweit wie möglich automatisieren. Auf Grundlage der integrierten Daten können wir mithilfe von statistischen Verfahren und maschinellem Lernen Informationen extrahieren, die für die untersuchten Krankheiten relevant sind. Die gewonnenen Informationen werden dann weiter ausgewertet, um unser Verständnis der Erkrankungen zu verbessern und darüber hinaus Risikofaktoren zu entdecken und möglicherweise Heilungsmöglichkeiten zu finden.
Contact: Stefan Bonn
—
Handicap Activité Cognition Santé – HACS
Le thème central de notre équipe est le handicap avec pour retombée attendue l’inclusion sociale des personnes en situation de handicap dans les différents lieux de vie (la maison, l’école, le travail, la cité, etc.). Nos travaux s’intéressent aux personnes avec handicaps ou maladies chroniques de l’hôpital aux lieux de vie dans une approche inclusive. Nos recherches concernent un vaste champ de pathologies neurologiques : accidents vasculaires cérébraux, maladies génétiques, inflammatoires et infectieuses, traumatismes crâniens, maladies dégénérative, troubles du spectre autistique, etc.) mais aussi le vieillissement neuro-ordinaire.
Contact: Hélène Sauzeon
—
HeKA team: Digital health research for a learning health system
The objective of HeKA is to develop methodologies, tools and their applications in clinics towards a learning health system, i.e., a health system that leverages clinical data collected to extract agilely and reliably novel medical knowledge that, in turn, continuously improves healthcare. We rely on the availability of EHRs (Electronic Health Records), clinical trials, cohorts and other linked data to develop models for stratification and prediction with the potential of improving the precision and the personalization of treatments, and in turn the quality of healthcare.
With this objective, HeKA research activity follows 3 interdependent axes: (1) Patient phenotyping and representation learning, (2) Stochastic and data-driven predictive models for decision guiding, and (3) Designs of next generation clinical trials.
Contact: Sarah Zohar
—
Human Cell Atlas
The Human Cell Atlas programme aims to chart the properties of human cells, building a reference map of the human body that can be used to understand human health and to treat disease. Contributing to this work, led by Professor Neil Lawrence, this Human Cell Atlas project is creating a detailed single-cell and spatial atlas of embryos in late organogenesis. Its aim is to build an in-silico atlas of the human embryo. Its approach is to deploy machine learning approaches to large-scale dimensionality reduction, focusing on latent variable techniques such as GPLVM or variational auto-encoders.
Contact: Neil D. Lawrence
—
Improved Treatments of Acute Myeloid Leukaemias by Personalised Medicine
We will initially focus on a rare AML subtype, pure erythroleukaemia (PEL) to develop a methodological pipeline to elucidate pathological mechanisms and subsequently adapt it to other AML subtypes. There will be a translational approach based on AML samples as well as longitudinal data from completed clinical trials, which will provide a high quality source for big data analytics and mathematical modeling. We pursue early identification of responders to novel signaling targeted drugs. The subclonal architecture of individual AML patients and their signalling status are mapped by mass and flow cytometry and validated by quantitative proteomics. Quantitative multiomics and clinical data will be combined with methods for machine learning to develop AML classifiers. AML_PM develops pipelines for clinical decision-making that enable next generation diagnostics for AML and tailoring treatment for individual AML patients.
Contact: Bjørn Tore Gjertsen
—
Improving the detection of relevant adverse outcomes through AI-based triage in the emergency department
The aim of the research is to produce a priority triage algorithm based on machine learning trained on relevant outcomes that have been scientifically evaluated within the scope of the thesis. The triage algorithm will be based on basic health data, patient experience, nurse intuition and will try to bring together advanced nursing assessments and high technology.
Contact: André Johansson
—
Improving Treatments in Cerebral-Palsy Children Using Artificial Intelligence
Within this project, we propose to develop a prototype software application for automatic analysis and evaluation of 3D motion data using sophisticated technologies employing artificial intelligence.
Contact: Ladislav Plánka
—
Integrative Datenanalyse und -interpretation. Generierung einer synaptisch-integrativen Datenstrategie (SYNIDS)
Wir werden die anfängliche Analyse von transkriptomischen und proteomischen Daten bereitstellen und deren Integration, um Schlüsselproteine, Netzwerkhubs und relevante Verkettungen zu detektieren. Wir werden uns auch mit einer weiteren Herausforderung dieses Sonderforschungsbereiches beschäftigen indem wir die Synaptische integrative Datenstrategie (SynIDs) entwickeln. Diese wird beim Erstellen kausaler Zusammenhänge unterstützen. SynIDs wird den Forschern auch erlauben die Ergebnisse aus verschiedenen Bereichen abzurufen und sie mit öffentlich zugänglichen Daten zu kombinieren. Dadurch wird es vereinfacht Hypothesen aufzustellen, die dann experimentell validiert werden können.
Contact: Stefan Bonn
—
Intelligent Imaging
This programme aims to change the way medical imaging is currently used in applications where quantitative assessment of disease progression or guidance of treatment is required. Imaging technology traditionally sees the reconstructed image as the end goal, but in reality it is a stepping stone to evaluate some aspect of the state of the patient, which we term the target, e.g. the presence, location, extent and characteristics of a particular disease, function of the heart, response to treatment etc. The image is merely an intermediate visualization, for subsequent interpretation and processing either by the human expert or computer based analysis. Our objectives are to extract information which can be used to inform diagnosis and guide therapy directly from the measurements of the imaging device.
Contact: Daniel Rueckert, Bernhard Kainz, Jose Caballero, Kanwal Bhatia, Kevin Keraudren, Ozan Oktay, Serge Vasylechko
—
Intelligent Spinal Biomechanics Research Centre
The Intelligent Spinal Biomechanics Research Centre (ISBRC) is a cross-faculty, cross-discipline and cross-institution collaboration dedicated to the enhanced assessment of spinal biomechanics using advanced imaging and data science techniques in the diagnosis of persistent spinal pain and disability.
The aim is to optimise the measurement of the biomechanics of the living spine using quantitative fluoroscopy (QF) and artificial intelligence (AI) to make biomechanical measurements possible in clinical practice.
Contact: Alan Breen & Marcin Budka
—
KÄVELI: Home Monitoring of Parkinson’s Disease
Käveli project builds a system to monitor and analyze the walking patterns of Parkinsons disease patients at home. Home monitoring is done using the sensors of the smartphone, force sensor integrated to smart insoles of shoes and wrist-worn accelerations sensors.
Contact: Jari Ruokolainen
—
Kernel Methods, Pattern Analysis and Computational Biology (KEPACO)
The KEPACO group develops machine learning methods, models and tools for data science, in particular computational metabolomics. The methodological backbone of the group is formed by kernel methods and regularized learning. The group particularly focusses in learning with multiple and structured targets, multiple views and ensembles. Applications of interest include metabolomics, biomedicine, pharmacology and synthetic biology.
Contact: Juho Rousu
—
KONSENS-NHE
The researchers are developing a brain-controlled hand exoskeleton that can be used in everyday life, enabling paralysed people to grasp everyday objects and thus live more independently. Dr. Surjo Soekadar, head of the Applied Neurotechnology research group at the University Hospital of Tübingen and project coordinator, is sure that this will substantially improve the quality of life for paralysed persons.
Contact: Surjo R. Soekadar
—
Learning Interpretable Models for Medical Diagnostics – DiagnoLearn
A central problem in practical use of statistical models is the interpretability of a model. In many applications it is quite useful to construct a scoring system which can be defined as a sparse linear model where coefficients are simple, having few significant digits, or are even integers. Ideally, a scoring system is based on simple arithmetic operations, is sparse, and can be easily explained by human experts. In this project, we challenge the problem of automated interpretable score learning purely from data.
Contact: Nataliya Sokolovska
—
Machine Intelligence From Cortical Networks (MICrONS)
Although neuroscience has inspired many elements of artificial neuronal networks, the mammalian visual system is still markedly different from current state-of-the-art deep neural networks in terms of its circuit architecture, robustness, and ability to learn. Two groups at the Bernstein center (Bethge, Sinz) are part of a multi-university consortium funded by the MICrONs program within the Obama BRAINinitiative, that is setting out to narrow the gap between current state-of-the-art deep learning and algorithms of the mammalian visual system by exploring circuit level functional and anatomical patterns of populations of cortical neurons.
Contact: Matthias Bethge
—
Machine Learning, Deep Learning & Neurosciences
Machine learning and deep learning are powerful tools for analyzing and modeling data from neuroscience experiments in order to answer specific questions. All the work to be done to push forward the research in the field of the ILCB within this QT is grouped in three axes. The first axis is about learning from data, Machine Learning and Deep Learning. The second point concerns the design of machine learning systems for brain data. The third axis focuses on the comparison of mental representations and computer representations.
Contact: Thierry Artières & Pascal Belin
—
Machine Learning in Biomedicine
Our team develops novel machine learning methods and models to answer key questions in biomedicine: how do mutations arise and contribute to disease? How to accurately predict cancer patient outcomes? What is the role of inherited genetic factors in diseases? Together with our collaborators, we focus on answering these questions in cancers and hematological malignancies. We create scalable and multimodal machine learning techniques utilizing genome, transcriptome, epigenome and imaging data to build clinically useful computational tools.
Contact: Esa Pitkänen
—
Machine Learning Modelling for AI-Guided Drug Response Prediction
We are making use of network pharmacology approaches to map target addictions and other dependency mechanisms that underlie individual drug sensitivity profiles, with the aim to identify synergistic drug-target combinations that can effectively inhibit multiple cancer driving sub-clones and other escape routes of cancer cells.
Contact: Tero Aittokallio
—
Machine Learning pour l’Aide au Diagnostic de l’Autisme chez l’Enfant via le « Eye Tracking »
Notre ambition : renforcer et structurer les équipes autour de la robotique chirurgicale, pour être en capacité de proposer des solutions innovantes permettant une optimisation du parcours, une médecine individualisée et des actes opératoires sécurisés et fiabilisés. Cette démarche implique de poursuivre l’évaluation des outils créés et enrichir ces systèmes en y intégrant le « Big data / intelligence artificielle ». Ces techniques auront, à coup sûr, des impacts forts sur le parcours de soin (imagerie, assistance robotisée, outils d’aide à la planification et à la réalisation de l’acte opératoire, domotique)
Contact: Michel Lefranc
—
MAGIcIAN
Antimicrobial resistance (AMR) is increasing worldwide, and surveillance activities play a key role in informing policies to contain AMR. Moreover, resistance to new antibiotics is emerging ever quicker after their introduction onto the market, rapidly reducing the effectiveness of even last-resort antibiotics. As such, the sustainable introduction of a novel class antibiotic can only be achieved when accompanied by timely and informed surveillance and stewardship strategies.
Contact: Natacha Berbers
—
Maschinelles Lernen in der Laboratoriumsmedizin
Das medizinische Labor der Zukunft wird neben dem Erstellen von Befunden auf höchster Qualität eine zunehmend interaktive Ausrichtung annehmen. Dabei erhalten klinisch tätige Kolleginnen und Kollegen Empfehlungen zu Stufendiagnostik und Diagnosefindung, die auf Basis der Messwerte ermittelt werden. Um dieses Ziel zu erreichen, werden in unserer Arbeitsgruppe moderne Methoden zur Datenauswertung angewendet, wie z.B. nicht-lineare, multiparametrische Machine Learning Methoden mit deren Hilfe klinisch relevante Diagnosen vorhergesagt werden sollen. Hohe ethische Vorgaben und Datenschutzregularien (European General Data Protection Regulation GDPR / DSFVO) werden dabei berücksichtigt.
Contact: Amei Ludwig & Claas Schmidt
—
Medical AI: Developing, evaluating and deploying machine learning in healthcare
Medical AI deals with the application of artificial intelligence, primarily machine learning and data science, to problems in healthcare. As a field, it primarily lies in the intersection of clinical medicine, medical research, and machine learning engineering. Our activities are therefore based on a close collaboration between medical researchers from UiB, software engineers and machine learning engineers from HVL, and medical professionals in Helse Bergen and Helse Vest. Our mission is to contribute to an increased degree of personalized medicine and better decision support for diagnosis, prognosis, and therapy. Especially in diseases and conditions where images are an important source of information.
Contact: Arvid Lundervold
—
Medical Image Processing
We develop new algorithms for biomedical image processing. We process images from different modalities, such as magnetic resonance, ultrasound, computed tomography, or microscopy. We work in 2D, 3D and 4D. We know how to preprocess the data, how to register, segment, model, reconstruct and classify them. We use techniques from image processing, numerical mathematics, as well as machine learning.
Contact: Jan Kybic
—
Medical Machine Learning Lab
Mental disorders are among the most debilitating diseases in industrialized nations today. […] [Valid] predictive models would be instrumental, both, for minimizing patient suffering and for maximizing the efficient allocation of resources. Realizing this potential is the core goal of this research group. To this end, we employ state-of-the-art tools from machine learning, artificial intelligence and statistical learning such as Deep Neural Networks, Random Forests etc.
Contact: Tim Hahn & Dominik Grotegerd
—
MIRACUM
The goal is to take advantage of digitalization in medicine, to link data and generate medical knowledge, and to develop and apply innovative IT solutions for a better, data-based healthcare delivery system.
Contact: Hans-Ulrich Prokosch
—
Mit künstlicher Intelligenz Krebs gezielt behandeln
Zuerst wollen die Forscher eine große Datenbank erstellen. Darin werden die histologischen Bilddaten und umfangreiche molekulare Daten von jeweils 1.000 Fällen häufiger Tumoren, wie Lungenkrebs, Darmkrebs und Bauchspeicheldrüsenkrebs, enthalten sein. In einem nächsten Schritt soll ein computergestütztes System darauf trainiert werden, auf Grundlage der histologischen Bildern wichtige molekulare Gruppen vorherzusagen. Falls dies mit ausreichender Genauigkeit gelingt, könnte das System in Zukunft dazu eingesetzt werden, diejenigen Tumoren schneller und kostengünstiger zu identifizieren, die besonders gut für eine bestimmte „Schlüssel-Schloss-Therapie“ geeignet sind.
Contact: Philipp Ströbel
—
Modeling and Development of Personalised Medicine
Work by Pietro Lio uses machine learning approaches to analyse bio-medical “big data” for disease modeling and development of personalised medicine, with integration across scales from the molecular and genomic to organ and systems levels.
Contact: Pietro Lio
—
Modeling for Neuroimaging Population Studies
Population imaging relates features of brain images to rich descriptions of the subjects such as behavioral and clinical assessments. We use predictive analysis pipelines to extract functional biomarkers of brain disorders from large-scale datasets of resting-state functional Magnetic Resonance Imaging (R-fMRI), Magnetoencephalography (MEG) and Electroencephalography (EEG). We also built tools for automated data analysis which facilitate processing large datasets at scale. Some of our results are highlighted below.
Contact: Bertrand Thirion
—
Multitasking Learning Method Using Deep Neural Networks: Application to the Identification of Respiratory Suffering
The aim of this thesis is to design robust automatic facial expression analysis methods that rely on transfer learning and multitasking to identify, characterize, and monitor respiratory discomfort in the absence of direct human interaction. This study provides long-term prospects for innovative applications such as the automatic monitoring of intubated patients or the design of intelligent ventilators that can adapt to the patient’s sense of discomfort.
Contact: Gérard Biau
—
NemoPlast: Learning with Neurorobots: Human-Machine Interfaces for the Promotion of Motor Plasticity
In the project „NemoPlast: Learning with neurorobots: human-machine interfaces for the promotion of motor plasticity“, scientists led by Professor Alireza Gharabaghi […] are developing a novel training system for stroke patients; many of them are still significantly restricted in their motor skills years after the event. For these patients, NemoPlast is developing a training neurorobot that links an exoskeleton with a non-invasive brain stimulator.
Contact: Alireza Gharabaghi
—
NeuroControl: Control of Physiological Activity in Retinal Neuronal Networks
The project „NeuroControl: Control of physiological activity in retinal neuronal networks“ focuses on how activity in the neuronal networks of the retina can be controlled.
Contact: Philipp Berens & Günther Zeck
—
Neuroimaging methods group (NIMEG)
We work at the intersection of neuroscience and neurotechnology by developing novel measurement and analysis methods for studying human brain structure and function, as well as applying these methods to address important and challenging research questions in both basic and clinical neuroscience. We extensively employ neuromagnetic measurements of brain activity, which give a temporally detailed picture of activation dynamics.
Our aims are: to substantially improve the spatial resolution of neuromagnetic measurements by new instrumentation as well as by physiologically-informed computational modeling; to develop novel analytical approaches for neuroimaging data, also for real-time use to support closed-loop experiments where the subject’s brain activity affects subsequent stimulation – in particular, we focus on assessing functional connectivity between brain regions and between the brains of interacting subjects; to investigate the neural processes supporting cognitive functions such as attentional selection, conscious perception, mental imagery and metacognition.
Contact: Lauri Parkkonen
—
Neuroinformatics Group
We apply machine learning models on neuroimaging data, in particular MEG. We model the visual system in the brain by analyzing the statistical structure of the natural input images. We develop the relevant theory of statistical machine learning, typically unsupervised.
Contact: Aapo Hyvärinen
—
ONCOTYPE-URO
Le GRC n°5 est une équipe transdisciplinaire orientée sur les cancers urologiques (prostate, rein, voie excrétrice urinaire). Ses activités reposent sur l’analyse de bases de données clinico-biologiques, génétiques et moléculaires afin d’intégrer les différentes composantes phénotypiques ou génotypiques dans des modèles prédictifs applicables aux situations cliniques de prévention, de diagnostic, de pronostic et de théranostic. Les méthodes de modélisation utilisent l’intelligence artificielle explicable (XAI) pour générer des outils d’aide à la décision applicables en clinique.
Contact: Olivier Cussenot
—
Patient-Centric Engineering in Rehabilitation (PACER)
In the western world, about 50% of major amputations is caused by diabetes, and the majority of these have had a long and complex medical history. They have often been too inactive, had pain and impaired general condition. It is critical that these people are motivated to take back control of their lives, and assisted to increase their quality of life. We believe that a device that could follow the patient will facilitate for a personalized and optimized rehabilitation. To the best of our knowledge we have not found any attempts to apply such scoring rules on machine learning, artificial neural network or deep learning models in a personalized and optimized device for rehabilitation of amputated patients.
Contact: Peyman Mirtaheri
—
PEDIA
The aim of the PEDIA study is to investigate the value of computer-assisted analysis of medical images and clinical features in the diagnostic workup of patients with rare genetic disorders.
Contact: Tzung-Chien Hsieh
—
Personalised Disease Modelling
This project aims to use state-of-the-art Artificial Intelligence techniques in order to learn the “shape” of disease as it progresses. This will enable models to be built that capture realistic progression for an individual patient, facilitating better management of disease and more appropriate interventions.
Contact: Allan Tucker
—
PET/MRI in gynecological tumors
The subject of the current studies is an evaluation of whole-body PET/MRI as a possible alternative to PET/CT in cases of suspected recurrence. In addition, an evaluation of PET/MRI for primary spread diagnosis of advanced cervical and vulvar carcinomas is performed. Initial results on radiomics analysis of multiparametric PET/MRI propagation diagnostics in primary cervical carcinomas show the high predictive value of specific parameters. These aspects of radiomics and machine learning-based analysis of multiparametric PET/MRI datasets is the subject of ongoing studies.
Contact: Lale Umutlu
Website (in German)
—
Predicting Alcohol Use Disorder through Machine Learning
We aim to solve the public health conundrum of risk-stratification for alcohol use disorder by means of the method of machine learning. Machine learning searches the best solution for a given problem in a data-set and it bypasses the need to selectively study single options. That is, machine learning is not restricted by background theory or human biases. The product of machine learning is a set of rules or contingencies that serve to implement, individual, risk stratification of alcohol use disorder. Now we have the expertise to apply this method on the rich and well defined data from the Netherlands Study of Depression and Anxiety.
Contact: Marc Molendijk
—
Predicting Medication Response in ADHD through Computational Modeling of the Continuous Performance Test
The current project takes a novel approach to prediction of medication response. By using computational modeling of decision making we will analyse already collected data from 250 adult ADHD patients.
Contact: Mads Lund Pedersen
—
Prediction and Decision Support Systems for Knee Osteoarthritis
Osteoarthritis (OA) is the most common joint disease in the world. Despite the extensive research, the etiology of OA is still poorly understood and its progression is highly difficult to predict clinically. However, large amount of accumulated clinical and research data exists, which enables new possibilities to understand OA progression when analysed with novel machine learning based methods.
Contact: Simo Saarakkala
—
Prediction of Epileptic Seizures from Multivariate iEEG Recordings
Using machine learning to predict and control epileptic seizures in drug-resistant patients.
Contact: Lorenzo Livi
—
R&D of Innovative Technology for Predicting and Early Warning of Delayed Cerebral Ischemia after Subarachnoid Hemorrhage (EWoDCI)
Project aims: to develop an innovative method for predicting and early warning of CV and DCI after aSAH, to perform clinical studies of this method and to create a software tool for forecasting vasospasm and cerebral ischemia.
Contact: Vytautas Petkus
—
Radiomics and artificial intelligence in radiotherapy
Medical image data is a very important and comprehensive source of data that contains significantly more information than can be perceived by mere human observation. Through radiomics and artificial intelligence methods, this information can be used to individually improve radio and combined radio system therapies.
The newly founded research group has the innovative concept to explore the possibilities of radiomics and artificial intelligence in radiotherapy with a strong interdisciplinary focus.
Contact: Florian Putz, Yixing Huang, Christoph Bert & Benjamin Frey
Website (in German)
—
Remote Sensing and Advanced Spectral Analysis for Coaching and Rehabilitation
This research project is part of a series of activities carried out with Cambridge Centre for Sport and Exercise Sciences, using smart sensors/wireless sensors and audio analysis in biomechanics and biomedical sciences.
Contact: Domenico Vicinanza & Jin Zhang
—
RESPOND3 – Responsible Early Digital Drug Discovery
Using machine learning to tackle a computational bottleneck in the drug discovery and development process.
Contact: Nathalie Reuter
—
REVERT: Artificial intelligence in oncology
The EU-funded REVERT project will address at systems level the pathophysiology of advanced colorectal cancer in patients responding well or poorly to therapies, with the goal to design an optimal strategy for therapeutic interventions depending on patients‘ features. This goal will be achieved using a large number of standardised biobank samples and clinical databases from several clinical European centres, including known and new potential prognostic biomarkers. Following the AI-based data analysis, results will be evaluated for the REVERT-DataBase impact on survival and quality of life in prospective clinical trials. The project will also generate a broad network among industrial and academic partners focused on the development of personalised medicine.
Contact: Jenny Persson
—
Robotic Surgery
The University Robotic Centre consists of a specialized room equipped with the Da Vinci S HD robot, related technology and state-of-the-art monitors for mini-invasive robot-assisted surgeries.
Contact: Vladimír Študent
—
SCAMPI
SCAMPI’s primary objective is to co-design and develop a new intelligent computer-based toolkit that will help and support people affected by dementia and/or Parkinson’s in their daily living.
Contact: Neil Maiden
—
Sensor informatics and medical technology
Research of the group focuses to sensor informatics, adaptive signal processing, and data fusion systems especially for medical applications. For the computations, sensor informatics uses adaptive signal processing methods, machine learning, and statistical methods together with mathematical models of the physical phenomena. The used methods include Kalman filters, particle filters, Markov chain Monte Carlo (MCMC), Bayesian analysis, kernel methods, and non-linear classifiers among others. Our group has developed many advanced methods for biosensor signal processing. Currently, our group has expanded medical research in computed tomography (CT) imaging.
Contact: Simo Särkkä
—
SingleStatOmics
Massively parallel sequencing applied to single cells allows us to investigate new questions that were out of reach for classical bulk genomics. Cell-to-cell variability is central in gene regulation or cell differentiation, as it provides information on the underlying molecular networks. Consequently, single cell expression profiling has the promise of revolutionizing our understanding of genomes regulation.
Contact: Franck Picard
—
Smart Heart
We are aiming to develop an intelligent MR scanner that enables fast, efficient and effective diagnostic imaging. This will be achieved by combining advances in how MR images are acquired, reconstructed and analysed with advances in Artificial Intelligence (AI) and Machine Learning (ML).
Contact: Katherine Bellenie
—
Sonification and Smart Sensors for Healthy Ageing
This project will investigate the design and implementation of small (possibly wearable), wireleless smart sensors with a special focus on healthy ageing.
Contact: Domenico Vicinanza & Jin Zhang
—
SPRING: Socially Pertinent Robots in Gerontological Healthcare
In the past five years, social robots have been introduced into public spaces, such as museums, airports, commercial malls, banks, company show rooms, hospitals, and retirement homes, to mention a few examples. In addition to classical robotic skills such as navigation, grasping and manipulating objects, i.e. physical interactions, social robots must be able to communicate with people in the most natural way, i.e. cognitive interactions.
Contact: Xavier Alameda-Pineda
—
SUBSAMPLE Digiteo chair
The goal of the project is to understand the neurobiological mechanisms that are involved in complex neuro-psychological disorders. A crucial and poorly understood component in this regard refers to the interaction patterns between different regions in the brain. In this project we will develop machine learning methods to capture and study complex functional network characteristics.
Contact: Bertrand Thirion
—
Systems Biology of Drug Resistance in Cancer
The focus of the research group is to understand and find effective means to overcome drug resistance in cancers. Our approach is to use systems biology, i.e., integration of large and complex molecular & clinical data (big data) from cancer patients with computational methods and wet lab experiments, to identify efficient patient-specific therapeutic targets. We are particularly interested in developing and applying machine learning based methods that enable integration of various types of molecular data (DNA, RNA, proteomics, etc.) to clinical information.
Contact: Rainer Lehtonen
—
The Artificial Intelligence Clinician Learns Optimal Treatment Strategies for Sepsis in Intensive Care
[We] developed a reinforcement learning agent, the Artificial Intelligence (AI) Clinician, which extracted implicit knowledge from an amount of patient data that exceeds by many-fold the life-time experience of human clinicians and learned optimal treatment [for sepsis] by analyzing a myriad of (mostly suboptimal) treatment decisions.
Contact: Anthony C. Gordon
—
The Interpretation of Physical Activity Wearable Data and its Relation with Metabolic and Brain Health in Older Adults
Because the standard interpretation of the accelerometer data does not provide enough insight about the physical activity (PA) of the study participants in free-living conditions, further analysis to combine wearable and heath data requires an experienced data scientist. In the past two years we generated labelled activity data in a validation study of 35 older adults, using accelerometers and physiological sensors. Using this dataset and state of the art machine learning algorithms, together with LIACS we created multiple activity recognition models, which can be applied to free living data collections. We are now ready to interpret free-living physical activity profiles in the LUMC studies and combine them with health parameters data, such as MRI data on brain ageing and metabolic health measured by traditional clinical parameters and metabolomics.
Contact: Eline Slagboom
—
Understanding mental health with data science
Led by Dr Sarah Morgan, this group applies data science approaches to better understand mental health conditions, including machine learning, network science and NLP methods. A core area of focus is the use of MRI to study brain connectivity in schizophrenia and other mental health conditions. The group uses brain MRI to estimate brain networks, where nodes represent macroscopic brain regions and edges represent connectivity between regions. This allows exploration of whether connectivity patterns can be used to predict individual patients’ disease trajectories and what such patterns reveal about the biological mechanisms underlying mental health conditions, for example by relating brain MRI networks to genetic and genomic data. The group is also interested in using other data modalities to study mental health, with projects investigating the potential of transcribed speech data to predict risk for psychotic disorders and mapping transcribed speech excerpts as networks. Sarah’s research applies AI and data science approaches to better understand and predict brain development, cognition and mental health. To that end, she uses a range of methods from machine learning, network science and Natural Language Processing.
Contact: Sarah Morgan
—
Using Artificial Intelligence to Identify Biomarkers for Psychiatric Disorders
Ziel des Projekts ist es, objektive physiologische Marker für psychiatrische Störungen wie Schizophrenie-Spektrum-Störung (SSD) und Autismus-Spektrum-Störung (ASD) zu identifizieren. Mit Hilfe des Elektroenzephalogramms und der künstlichen Intelligenz wollen die Partner die gegebenen experimentellen Paradigmen optimieren, um visuelle Biomarker auf frühen Verarbeitungsstufen für SSD und ASD zu messen. Das Projekt wird von Eucor – The European Campus mit „Seed Money“ in der Förderlinie „Forschung und Innovation“ unterstützt.
Contact: Jürgen Kornmeier
—
Using Machine Learning to Identify Noninvasive Motion-Based Biomarkers of Cardiac Function
This project aims to apply state-of-the-art imaging, motion analysis and machine learning techniques to characterise the motion of the heart as it beats.
Contact: Daniel Rueckert & Wenjia Bai
—
Using Virtual Reality to Help Surgeons
Researchers at Bournemouth University (BU) have been working on technology that uses animation, special effects, artificial intelligence and virtual reality to help surgeons develop skills and prepare for surgery.
Contact: Xiaosong Yang
—
Weakly Supervised Learning for Accurate Annotation of Textual Clinical Documents
The extraction of medical concepts (diseases, signs, symptoms, treatments, drugs, etc.) from clinical reports is an important research topic in natural language processing. These documents, written in natural language, by humans and for humans, are still very difficult to analyse and therefore to valorise, due to the variation of language in general, but also to the technical nature of the documents, whose vocabulary varies strongly from one medical specialty to another.
Contact: Gérard Biau
Environmental Science
3D modeling of large-scale environments for the smart territory
We are exploring the generation of rich 3D vector maps with semantic attributes from raw measurement data. We plan to learn geometric priors and error metrics that locally adapt to the semantic class of objects. We are developing a pliant approach with the capability to model the wide range of objects, which abound in open environments of the smart territories.
Contact: Pierre Alliez
—
A Bird’s-Eye View on Agricultural Transformation in sub-Saharan Africa: An analysis of living standards indicators combining panel data, satellite imagery and deep learning
In this project we address longstanding and unresolved questions in development research regarding the distributional effects of rural transformations, poverty and production levels. We do this through an innovative framework featuring a new application of artificial intelligence techniques. More precisely, we apply machine learning to satellite imagery along with more conventional panel survey data. This is the first study of its kind and combines expertise from distant disciplines such as physics, development research and remote sensing in a cross-disciplinary effort.
Contact: Ola Hall
—
A Deep Learning-Based Automated System for Seabed Imagery Recognition and Quantitative Analysis (DEMERSAL)
The project consortium brings together specialists in signal, image and video processing, and marine benthic ecologists with long-term experience in UW research. We plan to develop a user-friendly system, flexible enough to use in a variety of marine environments. To test system’s capabilities, video material collected in the Arctic Ocean, Baltic Sea, Mediterranean Sea and other world regions will be used.
Contact: Anatanas Verkikas
—
A modular remote sensing pipeline incl. ground-truth monitoring for automated snow avalanche detection and forecasting
Recently, deep learning architectures have been applied to avalanche detection, leading to improved detection probability and accuracy. An operational avalanche monitoring system in Austria with state-of-the-art automatic detection and prediction based on satellite imagery could drastically improve avalanche forecasting and increase the safety of people, buildings and infrastructure in the long term. In the framework of RSnowAUT, we want to set up an automatic avalanche detection system for Austria based on Sentinel-1 SAR imagery and including a best-practice data pipeline and deep learning algorithms. We also want to set up a first test system for automated avalanche forecasting.
Contact: Stefan Muckenhuber
—
AI tools of Deep Learning to improve simulation of clouds and climate
Clouds have an important influence on the climate, they can both reflect sunlight back to space and reflect heat radiation back to Earth, besides being integral to weather in the form of rain and other precipitation. Cloud formation is also linked to other climate-related processes, such as, carbon dioxide (CO2) emissions. To model the climate, it is therefore important to get these balances right. Furthermore, the weather itself is affecting other areas, not the least renewable energy sources, such as, solar, wind- and hydro-power, which depend on sun radiation, wind speeds, and precipitation.
Common global models lack the resolution to treat clouds with much accuracy and more detailed physical models become cumbersome on this scale. Artificial intelligence (AI) in the form of machine learning has the ability to create representations of data that are more efficient to use as models once they have been obtained than to re-calculate the physical processes all the time.
The purpose of this project is to accelerate a detailed model of clouds with AI technology so as to acquire insights into climate change. The project will simulate the response of climate to CO2 emissions. This will enable an assessment of how climate change during the rest of this century will affect solar, wind- and hydro-power in northern Europe.
Contact: Vaughan Phillips
—
AquaImpact
AquaIMPACT aims to integrate the fields of fish breeding and nutrition to increase the competiveness of EU’s main aquaculture species while minimizing environmental impact.
Contact: Jochen Hemming
—
Artificial Intelligence and Landscape Analysis: Expanding Methods and Challenging Paradigms
In the last decade artificial intelligence has started assisting researchers in performing complex computational operations, providing scholars with the possibility to analyze datasets so far considered too large or too complex to be surveyed by humans. The exponential development and diffusion of new data-acquisition technologies, the expansion of the world wide web and the increased availability of large datasets of heterogeneous spatial geographic information, opened incredible opportunities for geo-scientists to explore and define novel investigation approaches. this project will develop an investigation approach based on the systematic training of highly intelligent machines for detecting scattered archaeological elements visible in different datase
Contact: Nicolo Dell’Unto
—
Artificial Intelligence for Retrieval of Forest Biomass & Structure
We present a new research project funded by Academy of Finland AIPSE program aimed at using advanced AI methods, a well-validated physically-based forest reflectance model, and EO data to map forests in the boreal zone. We will use the simulated spectra and the corresponding forest structural data to train AI algorithms; once trained, we will apply the algorithms to optical EO data from Sweden, Finland, Estonia and Russia, and hyperspectral data from Finland. The AI retrieval results will be compared against forestry data from test sites in each of these regions.
Contact: Jorma Laaksonen
—
Artificial Intelligence to improve the coupling between the Antarctic Ice sheet and the ocean/atmosphere system – AIAI
In this project, we aim to improve the integration of the Antarctic ice sheet into an Earth System Model through the use of neural networks at the coupling interfaces. These will bring increased resolution and account for polar processes absent or poorly represented in Earth System Models (e.g., surface melt and runoff, ice-shelf basal melt). Neural networks will be trained on high-resolution polar-oriented atmospheric and oceanic simulations, including in a warmer climate and with modified ice sheet geometry. Members of our consortium have recently conducted two pilot studies on neural networks that serve as proofs of concept for this project.
Contact: Nicolas Jourdain
—
Artificial probabilistic information for ocean-climate applications – REPLICA
REPLICA will apply statistical and machine learning techniques to a variety of oceanic data sets with the aim of improving our understanding of the impact of intrinsic ocean variability on climate change metrics, and a focus on the North Atlantic. This region is critical in determining European climate, but is strongly influenced by intrinsic variability through the Gulf Stream / North Atlantic Current. We will seek to do achieve this improved understanding by: (i) enhancing existing probabilistic information, and (ii) developing accessible and sustainable methods of obtaining probabilistic information that do not depend on direct numerical simulation.
Contact: Sally Close
—
Automated System of Geodetic Deformation Monitoring of Engineering Structures (ASGDM)
Automated system of geodetic deformation monitoring of engineering structures (ASGDM) is intended for the formation of a data bank, in order to ensure with the help of monitoring mode the control strain of engineering objects on the basis of integrated use of field methods of observation.
Contact: Galina Nikolayevna Tkacheva
—
Biosphere-atmosphere interactions of cryptogamic communities at the Amazon Tall Tower Observatory (ATTO) and their relevance across spatial scales
The Amazon basin hosts the largest contiguous tropical forest area and is a stabilising factor in the Earth’s climate system. Cryptogamic communities consisting of cyanobacteria, algae, fungi, lichens and mosses are ubiquitous, but there are few studies on the functional roles they play in atmospheric processes and biogeochemical cycles. Among other research methods, the classification and mapping of cryptogamic communities will be based on drone-based digital images classified by object-based image analysis using artificial intelligence supported by morphological and molecular identification methods.
Contact: Bettina Weber
—
Bridging geohysics and MachinE Learning for the modeling, simulation and reconstruction of Ocean DYnamics – MeLODy
Artificial Intelligence (AI) technologies and models open new paradigms to address poorly-resolved or poorly-observed processes in ocean-atmosphere science from the in-depth exploration of available observation and simulation big data. This proposal aims to bridge the physical model-driven paradigm underlying ocean & atmosphere science and AI paradigms with a view to developing geophysically-sound learning-based and data-driven representations of geophysical flows accounting for their key features (e.g., chaos, extremes, high-dimensionality). Upper ocean dynamics will provide the scientifically-sound sandbox for evaluating and demonstrating the relevance of these learning-based paradigms to address model-to-observation and/or sampling gaps for the modeling, forecasting and reconstruction of imperfectly or unobserved geophysical random flows. To implement these objectives, we gather a transdisciplinary expertise in Numerical Methods, Applied Statistics, Artificial Intelligence and Ocean and Atmosphere Science.
Contact: Ronan Fablet
—
CDE : Contrôle et Diagnostic pour l’Environnement
CDE est une équipe Toulonnaise dont les activités vont de la théorie du contrôle (contrôle optimal non linéaire, convergence des observateurs, etc.) aux aspects plus pratiques, incluant le diagnostic, en passant par différentes approches de l’automatique moderne (commande sans modèles, approches basées sur l’intelligence artificielle). Les applications concernent majoritairement l’environnement (énergies renouvelables, agriculture raisonnée, applications dans le domaine maritime).
Contact: Frederic Lafont & Nicolas Boizot
—
CENÆ: Compound Climate Extremes in North America and Europe: from dynamics to predictability
In CENÆ I aim to provide a step-change in our understanding of the drivers and predictability of compound climate extremes, and illuminate how climate change may affect these two aspects. I will specifically focus on two high-impact compound extremes which have occurred with an ostensibly high frequency in recent years: (i) wintertime wet and windy extremes in Europe; and (ii) same as (i) but with the additional occurrence of (near-)simultaneous cold spells in North America. CENÆ builds upon my ongoing contribution to developing dynamical systems analysis tools for climate extremes. It further leverages the work of my research group on the atmospheric circulation and machine learning for the study of atmospheric predictability. I will use this interdisciplinary knowledge base to elucidate the atmospheric precursors to compound extremes, provide a nuanced understanding of their predictability and point to new predictability pathways.
Contact: Gabriele Messori
—
Centennial Climate Drivers of Glacier Changes in Greenland
Uniquely well-documented glacio-meteorological observations from the famous Alfred Wegener Expedition (1929-1931, a remarkable warm period) and modern data obtained within WEG_RE, will allow us to quantify climate/glacier feedbacks on a centennial scale in West Greenland. The following research questions (Q) and hypotheses (H) guide us through WEG_RE: Q1: What are the typical spatial patterns of atmospheric conditions and ablation on a land-based Greenland outlet glacier? H1: The complexity of atmospheric conditions over a glacier surface determines ablation patterns. This complexity can be predicted depending on synoptic conditions and surface properties with deep-learning (DL) algorithms. Q2: How temporally constant is the relationship between atmospheric conditions and surface melt rates on a centennial scale? H2: Identical atmospheric conditions outside the glacier almost a century apart lead to different ablation rates at the fundamentally altered glacier surface. We hypothesize that these differences are a result of changed surface geometry and surface properties. Q3: To what extent does the quality of glacier reconstructions improve with well-constrained input on a centennial scale? H3: Glacier simulations with and without considering the time-varying relationship between atmospheric conditions and ablation are significantly different in their simulation result of glacier topography. The constrained simulations are better able to simulate earlier glacier conditions.
Contact: Jakob Abermann
—
Constraining the Large Uncertainties in Earth System Model Projections with a Big Data Approach
The project COLUMBIA is an interdisciplinary project that aims to develop an innovative tool, based on the state-of-the-art machine learning technology, to efficiently analyze large amount of model data to better understand
why some models behave very differently than the others.
Contact: Jerry Tjiputra
—
Data-driven modeling for sustainable mining
Flotation is the dominating process in the global copper, lead, and zinc mining industries to separate valuable minerals from waste material. In the upstream process steps, the ore is ground to liberate all mineral grains, and mixed with water to form a slurry. Today, the flotation process is typically controlled semi-manually, where simple control loops stabilize tank levels and flow rates, while operators adjust parameters like airflow, reagent- and lime- addition based on the available measurements and experience. Model predictive control solutions have been attempted, with some success. However, performance is severely limited by poor model accuracy and the inability to adapt to changes in ore properties as new areas of the mine are excavated. To increase efficiency and autonomy of mineral processing, these challenges must be addressed. In this project, we will therefore push the state-of-the-art within mining process control by complementing machine learning with physics-based models based on e.g., conservation laws.
Contact: Kristian Soltesz & Margret Bauer
—
Deep Neural Networks for Multi-Scale Modeling of Climate Data Dynamics
The general framework of the thesis is the development of hybrid systems combining physical modeling and statistical and neuronal modeling. The subject concerns the modeling of complex physical phenomena, which concern the dynamics of ocean circulation, which are components of climate models. The objective is to model dynamic systems, based on statistical models based on deep neural networks which integrate knowledge and constraints from the physics of the phenomenon. The subject requires in-depth skills in statistical learning and neural networks and an interest in climate modeling.
Contact: Marie Deschelle
—
Detection and attribution of regional-scale climate change by neural methods
The objective of the project is to explore the contribution of recent methods of statistical learning and deep neural networks to meet different challenges of detection/attribution (D/A) studies. The aim is to develop algorithms capable of operating at global and regional scales, taking into account the uncertainties of models and observations. We will rely on recent advances in the field of neural networks to study in particular the reduction of dimensionality, taking into account of local spatio-temporal dependencies for attribution, and the probabilistic modeling of dependencies between observations and simulations.
Contact: Constantin Bône & Guillaume Gastineau
—
Development and Applications of New Methods in Seismic Research
We develop and apply new methods in wide range to processing of seismic data, to seismic modeling and to interpretation and post processing of the models. Research interests include automatic seismic signal processing, classification of seismic sources and signals, improving location accuracy, combining and comparing different geophysical 3D models and extracting new information from existing models. We have a special interest in applying machine learning methods to problems in seismic research.
Contact: Timo Tiira
—
DYNI team for bioacoustics
The DYNI team conducts research aimed at detection, clustering, classification and indexing bioacoustic big data in various ecosystems (primarily marine), space and time scales, in order to reveal information on the complex sensori-motor loop and the health of an ecosystem, revealing anthropic impacts or new biodiversity insights.
DYNI continues to install its bioacoustic surveillance equipment throughout the world including, France, Canada, Caribbean islands, Russia, Madagascar etc. Its present projects study different problematics like the influence of marine traffic on marine mammals.
Contact: DYNI research group
—
G2Net – A Network for Gravitational Waves, Geophysics and Machine Learning
The rapid increase in computing power at our disposal and the development of innovative techniques for the rapid analysis of data will be vital to the exciting new field of Gravitational Wave (GW) Astronomy, on specific topics such as control and feedback systems for next-generation detectors, noise removal, data analysis and data-conditioning tools.The discovery of GW signals from colliding binary black holes (BBH) and the likely existence of a newly observable population of massive, stellar-origin black holes, has made the analysis of low-frequency GW data a crucial mission of GW science. The low-frequency performance of Earth-based GW detectors is largely influenced by the capability of handling ambient seismic noise suppression. This Cost Action aims at creating a broad network of scientists from four different areas of expertise, namely GW physics, Geophysics, Computing Science and Robotics, with a common goal of tackling challenges in data analysis and noise characterization for GW detectors.
Contact: Isabel Cordero-Carrión
—
HEKTOR – Heterogeneous Autonomous Robotic System in Viticulture and Mariculture
The main objective of the HEKTOR project is to provide a systematic solution for the coordination and cooperation of smart heterogeneous robots/vehicles (marine, land and air) capable of autonomously collaborating and distributing tasks in open unstructured space/waters.
Contact: Nikola Mišković
—
High-Performance Processing Techniques for Mapping and Monitoring Environmental Changes from Massive, Heterogeneous and High Frequency Data Times Series – TIMES
The objective of the TIMES project is to produce new knowledge on the dynamics landscape objects from the massive exploitation of this big geospatial data with the objective to develop and validate novel data processing and analysis methods for environmental monitoring of landscape objects.
Contact: Anne Puissant
—
Impacts of DEep submEsoscale Processes on the ocEan ciRculation – DEEPER
The goals of the DEEPER project are (1) to quantify the impacts of deep-sea submesoscale processes and internal waves on mixing and water mass transformations, (2) to explore ways of parameterizing these impacts using the latest advances in machine learning, i.e. applying deep learning to the deep ocean.
Contact: Jonathan Gula
—
Improving Safety at Sea by Predicting Waves and Quiescent Periods
This project is using machine learning methods to model the sea’s surface from maritime radar observations, and then using the model to make predictions of that surface for up to two minutes into the future.
Contact: Jacqueline Christmas
—
InnovaMare – Blue Technology – Developing Innovative Technologies for Sustainability of Adriatic Sea
InnovaMare project will jointly develop and establish an innovation ecosystem model in the area of underwater robotics and sensors for purposes of monitoring and surveillance sector with a mission-oriented on the sustainability of the Adriatic Sea.
Contact: Nikola Mišković
—
KLIMOD – Computer Model of Flow, Flooding and Spread of Pollution in Rivers and Coastal Areas
The project carries out applied scientific research and develops a computer model for effective modelling of the flow and spread of pollution in open watercourses and in the coastal sea area, accepting river tributaries, torrents, and industrial and wastewater discharges into the coastal sea area. At the same time, a prediction model of microbiological pollution based on artificial intelligence models and the integration of microplastic models of pollution dispersion into the overall model will be developed. The computer model is adapted to the supercomputer environment, which enables high-resolution simulations to be carried out with the aim of implementing measures to mitigate the effects of climate change in priority vulnerable and transversal areas.
Contact: Vanja Travaš
—
Laboratory of Hydrological forecasting by Artificial Intelligence – Hydr.IA
The LabCom project associating the Hydrosciences Montpellier (HSM) research unit and the SYNAPSE company aims at developing a suite of hydrometeorological forecasting services based on artificial intelligence (AI) techniques. HSM is the leader research unit in the French environment for using artificial intelligence in hydrology whereas SYNAPSE is a SME specialized in the concentration and provision of hydrological data online. The range of the considered services brings solutions for the cases without any existing solution that would allow the users to anticipate phenomena to protect themselves better by any means. AI, by decreasing the costs of field studies, will enable providing reliable and affordable services for all the private and public water managers in order to significantly decrease the costs of flooding, which is the more impacting natural hazard.
Contact: Anne Johannet-Bretin
—
Land-ATmosphere Interactions in Cold Environments – LATICE
The research group LATICE will bring a focus on cold-regions exchange processes within Earth System Sciences as an interdisciplinary initiative of collaborative research and education.
Contact: Lena Merete Tallaksen
—
LEXIS-Project
The LEXIS project will build an advanced engineering platform at the confluence of HPC, Cloud and Big Data which will leverage large-scale geographically-distributed resources from existing HPC infrastructure, employ Big Data analytics solutions and augment them with Cloud services.
Contact: Jan Martinovic
—
LIVE: Phylogenetic and ecological determinants of fungi-algae-bacteria associations in lichens along latitudinal and elevational gradients
This research project aims at gaining a broad-scale view on fungus-algae-bacteria interactions along a latitudinal (southern Polar Regions) and elevational gradient (Alps). These gradients have overlapping climate conditions and will be compared. A novel perspective on community ecology with implications for climate change and its effects on southern South American, Antarctic and Alpine organisms will be provided.
Contact: Ulrike Waltraut Ruprecht
—
Machine Learning and Numerical Methods in Carbonate Geology
A large part of the group’s current research focuses on using artificial neural networks to automatically recognise and quantify rock features, such as facies, diagenetic fabric, and others.
Contact: Cédric M. John
—
Mapping of Algae and Seagrass Using Spectral Imaging and Machine Learning
The goal of the MASSIMAL project is to develop new methods for mapping underwater vegetation (seagrass and macroalgae). Using a hyperspectral camera mounted on a drone, the seafloor will be imaged from 50-100 meters above the sea surface. By combining the hyperspectral images with manual sampling of the vegetation, machine learning algorithms can produce detailed maps of e.g. the different species distribution, vegetation density and physiological state.
Contact: Martin Hansen Skjelvareid
—
MATS : Machine Learning for Environmental Time Series
A huge trend in recent earth observation missions is to target high temporal and spatial resolutions (e.g. SENTINEL-2 mission by ESA). Data resulting from these missions can then be used for fine-grained studies in many applications. In this project we will focus on three key environmental issues : agricultural practices and their impact, forest preservation and air quality monitoring. Based on identified key requirements for these application settings, MATS project will feature a complete rethinking of the literature in machine learning for time series, with a focus on large-scale methods that could operate even when little supervised information is available.
Contact: Romain Tavenard
—
Next Generation Biomonitoring of Change in Ecosystem Structure and Function
Our vision is to develop and test a generic NGB approach that will detect ecosystem-wide change more rapidly, sensitively and cheaply than current biomonitoring. Using a unique combination of Next-Generation Sequenced DNA data and Machine Learning, NGB will reconstruct species interaction networks to identify change in ecosystem properties, revolutionising both our understanding of ecosystems and our ability to predict and mitigate global change.
Contact: David Bohan
—
Predictive Model of the Synergistic Effects of Environmental Pollutant Mixtures
This project proposes to develop a unique fly-based model to predict synergistic interactions between mixtures of environmental pollutants, specifically on neuroendocrine signaling. We set up a very productive fly lab, complete with a range of methodologies, enabling for high-throughput metabolic and behavioral studies.
Contact: Helgi Schiöth
—
RainSolutions
There is a need to close the demand and supply gap in terms of quantity and quality of water resources. Therefore, the project “Research-based Assessment of Integrated approaches to Nature-based SOLUTIONS” (RainSolutions) aims to develop an integrated framework of methodologies to manage nature-based solutions (NBS) for the restoration and rehabilitation of urban water resources systems.
Contact: Miklas Scholz
—
SCAI Abu Dhabi & TOTAL Industrial Chair of Research on Artificial Intelligence
The project’s goal is to radically enhance the simulation of flow through porous media application domain in order to boost forecasting capabilities to be able to increase oil recovery and production. The modeling of fluid dynamics in porous media will be addressed by focusing on improving learning mechanisms using different perspectives, from learning the form of differential equations from data to learning dynamical models with limited computing complexity.
Contact: Gérard Biau
—
Seismic Imaging of the Earth Laboratory
The main research focus of the laboratory is the understanding of the processes inside the Earth by creating algorithms, collecting data and building seismic models. Identification of the geological scenarios for various structures is an important task that will help in solving many scientific and applied problems, such as mineral exploration, engineering applications, identifying sources of volcanic activation, understanding the mechanisms behind the emergence of mountains and valleys.
Contact: Ivan Kulakov
—
Territorial Security through environmental risks management
This project deals with risk assessments related to environmental extreme events. Analyses and predictions of floods, summer heatwaves, and storms are significant questions facing statisticians and risk assessors. Such environmental risks are the result of a long chain of casualties, involving several aleas, often correlated, with complex spatio-temporal dependent structures among extremes.
Our contributions in the prevention and management of environmental risks, will be twofold: 1/ Proposing novel and realistic definitions of risks indicators in environmental contexts. 2/ Studying in-depth their statistical inference, i.e. specifying more accurately the associated uncertainties.
In this project, the skills required to handle the modeling of these uncertainties are stochastic processes and random fields, spatio-temporal models, multivariate extreme theory, as well as practical expertise on spatial and environmental data gathered from firms in 3IA Côte d’Azur.
Contact: Elena Di Bernardino
—
The Framework for Evaluation of Crater Detection Algorithms
The results of this project are: (1) a method for crater detection using edge detection and gradient, modified Hough transform, morphometry measurements and analysis of topography and parameter space of Hough transform, slip-tuning, and calibration; (2) an improvement of crater detection utilizing a Crater Shape-based interpolation method that proved to be efficient for the detection of very small craters from the topographies of Mars and the Moon; (3) the framework for evaluation of crater detection algorithms (FECDA) including some of the most complete publically available crater catalogues.
Contact: Sven Loncaric
—
Using unmanned aerial vehicles and artificial intelligence to quantify ant impact on the Arctic ecosystem carbon dynamics
This project aims to increase our understanding how wood ants will influence carbon storage in soil and how climate change will affect their distribution in Arctic ecosystems. This project builds on detailed studies of ants, soil and vegetation interactions in mountain heaths and mountain forests in the Abisko region. The results will further be scaled to larger areas using images from drones and artificial intelligence. In detailed field studies we will measure how ants affect soil and vegetation parameters along an elevantion gradient.
Contact: Matthias Siewert
—
USMILE: Understanding and Modelling the Earth System with Machine Learning
In recent years, the volume of data from high-resolution models and observations has substantially increased to petabyte scales. Concomitantly, the field of machine learning (ML) has quickly developed, promising breakthroughs in detecting and analysing non-linear relationships and patterns in large multivariate datasets. Yet, traditionally, physical modelling and ML have been often treated as two different worlds with opposite scientific paradigms (theory-driven versus data-driven). Thus, despite its great potential, ML has not yet been widely adopted for addressing the urgent need of improved understanding and modelling of the Earth system. USMILE will combine multi-disciplinary expertise in ML and process-based atmosphere and land modelling to completely rethink model development and evaluation. ML will further allow us to define novel observational constraints on Earth system feedbacks and climate projections.
Contact: Veronika Eyring
Sociology
AI and big data policing for urban safety: new quantification regimes, market diversification, and reconfiguration of crime prevention – IAAP
More and more Artificial Intelligence (AI) innovations affect key social activities, such as policing. Smart CCTV, facial recognition, or predictive cartography are supposed to help us to build «safe cities». Beyond promises conveyed by such innovations, this research project aims to measure the concrete effects of AI on police work.
Three workpackages organize the research work. First, social scientists of the research team will conduct interviews and observation of the main actors from our four case studies. It is important to grasp how AI innovations transform police organization and activity : which part of the police work is automated, and how does it affect professional identities ? Secondly, we will objectify the relationships between scientific, industrial and police actors, through the observation of professional shows and exhibitions, and a network analysis. Finally, computer scientists of the research team will use the sociological results as a non-numerical data in order to correct and complete numerical data (coming from police records or captors such as CCTV). Building on XAI approach (eXplainable Artificial Intelligence), the project aims to better contextualize data and identify possible biases. The project will improve police reflexivity concerning its own activity, by designing an AI model based on collaborative, unbiased and explainable machine learning.
Contact: Florent Castagnino
—
CoCi: Co-Evolving City Life
The main questions of the CoCi proposal are: How could more participatory smart cities work, and how can they meet the requirements of being more efficient, sustainable and resilient? What are their risks and benefits compared with centralized approaches? How could digital societies fitting our culture, for example, based on values such as freedom, equality and solidarity (liberté, égalité, fraternité) look like, and what performance can be expected from them? The CoCi proposal brings together two research directions: first, the automation of mobility solutions based on the Internet of Things and Machine Learning approaches, as they have been pursued within the “smart cities” paradigm and, second, novel collaborative approaches as they have been recently discussed under labels such as participatory resilience, digital democracy, City Olympics, open source urbanism, and the “socio-ecological finance system”.
Contact: Dirk Helbing
—
Diagnostic System for Attitude Measurement Based on Psychological Distance Testing with a Use of Evolution Algorithms
The project aims to standardize and extend the innovative method of measuring pupil attitudes, interests and relationships for wide use in school and educational – psychological counseling. The project responds to the growing societal need for the development of the educational system, accompanied by the demand for current methods of diagnosing educational reality.
Contact: Lenka Skanderová
—
Distributed Artificial Intelligence for Collective Decisions in Smart Cities
Can you envision a more inclusive and direct democracy for our digital society empowered by an ethically-aligned AI and blockchain? This project will study and develop decision-support systems using blockchain and distributed AI for multi-agent systems, and will apply these to mobile crowd-sensing platforms and digital voting systems to empower trustworthy collective decisions in Smart Cities, as well as to understand collective crowd behavior.
Contact: Evangelos Pournaras
—
Generating Counter Arguments to Fight Disinformation on the Web – ATTENTION
There is a need to design intelligent solutions to fight the spread of disinformation in a pedagogical way, to persuade the user to stop the viral spreading of false information by providing verified counter-arguments.
In the ATTENTION project, we propose to address that urgent need by designing intelligent ways to identify disinformation online and generate counter-arguments to fight the spread of such information online. The idea is to avoid the undesired effects that come with content moderation when dealing with online disinformation, such as overblocking, and to directly intervene in the discussion, (e.g., Twitter threads) by engaging with people spreading incorrect information, through textual arguments that are meant to counter the fake content as soon as possible, and prevent it from further spreading. The project tackles this issue from a multidisciplinary perspective including law, sociology and Artificial Intelligence in order to ensure as a result AI solutions compliant with the ethical and sociological challenges connected to online disinformation.
Contact: Serena Villata
—
INEQUALITREES: A Novel Look at Socio-Economic Inequalities using Machine Learning Techniques and Integrated Data Sources
The INEQUALITREES project aims to investigate the levels and main drivers of two key manifestations of socio-economic inequality across the globe: poverty and inequality of opportunity (IOp, hereafter). We adopt a multidimensional, interdisciplinary and cross-national approach, by analysing IOp and poverty in three key individual outcomes (education, income and health) in four countries (Bolivia, Germany, India, Italy), and integrating contributions from economics, sociology, geography and computer science. We will look not only at how different socio-economic conditions shape life opportunities across the countries, but we will also map in detail within-country variations in socioeconomic inequalities. A key innovative feature of our project consists in the application of cutting-edge machine learning techniques to integrate and analyze large scale datasets from various sources, including national and international surveys, administrative and register data, as well as innovative data extracted from satellite images. Our research design will address common statistical problems in previous studies on IOp and poverty, such as small sample sizes, partial geographical coverage, and missing information on key variables. The combination of various data sources promises significant progress to understand the environmental and institutional features that countervail the existence of unfair socio-economic inequalities.
Contact: Moris Triventi
—
Instagram through the Prism of Artificial Intelligence
The transformations linked to digital technology are technical, but also epistemological, cognitive and finally social, economic and political. Within the framework of this doctoral research project, Instagram is the main field to apprehend Artificial Intelligence with a problematic angle questioning the calculated textualization of social practices by the digital device.
Contact: Gérard Biau
—
Legal and societal implications of Generative Machine Learning, aka ‚Creative Artificial Intelligence‘
CRE-AI is a postdoc project on the legal and societal implications of so-called creative or generative Artificial Intelligence, notably Generative Adversarial Networks (GANs). By blurring the distinction between fake and real (‚deepfakes‘), and its capacity to compose convincing new variations on existing patterns, generative AI challenges what we can believe to be true, what creativity is, and the security of any system running on AI. CREA-AI explores the perils and promises of the recent breakthroughs in the field of generative AI. The project also looks at how the outputs of (unsupervised) generative AI could impact on fairness, accountability and transparency problems arising from (supervised) classificatory AI.
Contact: Katja de Vries
—
NASTAC: Nationalist State Transformation and Conflict
Scholars studying contemporary conflict and development have increasingly turned to the historical roots of state formation. Going beyond Tilly’s classical theory of state formation, the NASTAC project creates a new theory of nationalist state transformation that will be evaluated with historical maps and archival data extracted through machine learning. While much has been written about state formation and nationalism, there is currently no empirically verified theory that shows how nationalism transformed and keeps transforming state internal and external properties, and how post-nationalist mechanisms counteract this influence. Without such a framework it is difficult to know under what conditions partition or power sharing should be used to pacify conflict-ridden multi-ethnic states.
Contact: Lars-Erik Cederman
—
Pandemotions
Maciej Gruszczyński, Daniel Popek, Dawid Rusiecki, and Marcin Wątroba are the team that analysed over 300 thousand tweets in terms of mood and expressing emotions during the pandemic. They categorised their content as neutral, motivating or expressing optimism, helplessness, derision, fear, or anger. The machine learning algorithms we use help to detect emotions among Twitter users. We use five models, three of which are deep neural networks – explain the students.
Contact: Przemysław Kazienko
—
Trust in Artificial Intelligence
To address this challenge, the current project focuses on trust—the critical building block of any society. The main goals of the project are to: (a) discern the differences between trust in humans and in AI agents; (b) identify the key psychological factors that determine the development of trust towards AIs; (c) determine the role of moral and competence components in the perception of AI (vs. human) trustworthiness; and (d) compare the relative weights of deeds and their consequences when making moral judgments about AIs (vs. humans).
Contact: Katarzyna Samson
Economics
AI in retail logistics
In the near future, there will be a transition from person-centred decision-making to more data-centred and automated decision-making. With AI/machine learning, we can today develop self-learning and self-optimising software, which automates data analysis and improves the decision-making. To realise the potential and obtain the benefits, a lot of new knowledge is needed. In logistics and retail, there are great opportunities to start utilising new technology and analyses better to respond to customers‘ and businesses‘ requirements for products and services.
Contact: Yulia Vakulenko
—
AI Predictive Analytics
This sub-area operates across a number of fundamental fields of AI methodologies. In Natural language processing (NLP) the focus is on how to extract information from large corpus of texts (such as media and social media) to explain the economy. Methodologies include: content analysis, concept extraction, document classification: Latent Dirichlet allocation (LDA), Multi-Label Classification. Research is also carried out using Causal Inference from Machine Learning approaches. The emphasis here is on methodologies such as Causal Bayesian Networks and Causal Random Forest to draw reliable conclusions from machine learning testing approaches. There is also focus on Deep Learning through the application of deep learning methods to analyze, predict and examine risk in real systems.
Contact: Amir Sadoghi
—
Algorithms for Systemic Risk Measurement
The goal of the project is to develop statistical methods for modelling and measuring systemic risk in complex systems such as financial markets. By researching the methods of mathematical and computational modeling, valuable insights can be obtained. For instance, the emergence of power-law and two-phase behavior in the financial market fluctuations, or the basic mechanisms that underlie systemic risk and the stability of the complex financial systems.
Contact: Zvonko Kostanjčar
—
An Intelligent Assistant Providing Risk Diagnosis for Industrial Procedures – LELIE
The main goal of this project is to produce software based on language processing and artificial intelligence that detects potential risks of different kinds (health, ecological, economical, etc.) in technical documents. We will concentrate on procedural documents which are, by large, the main type of technical document. Given a set of procedures (e.g. production launch, maintenance) over a certain domain produced by a company, and possibly given some domain knowledge (ontology or terminology), the goal is to process these procedures and to annotate them wherever potential risks are identified. Procedure authors are then invited to revise these documents.
Contact: Patrick Saint-Dizier
—
Artificial Intelligence for Digitizing Industry
Artificial intelligence plays a central role in societies, economies and industries around the world. However, there has been a lack of AI integration in Europe. As a result, potential users are not sufficiently supported despite the benefits it can provide to all branches of the industry and its digitisation. The EU-funded AI4DI project aims to transfer machine learning (ML) and AI from the cloud to the digitising industry. It will use a seven-key-target approach to evaluate and improve its relevance within the industry. The project plans to connect factories, processes and devices within the digitised industry by utilising ML and AI. It will then collect data on their performance.
Contact: François Alin (u.a.)
—
Artificial Intelligence Solution for Optimizing Companies’ Social Media Campaigns (EMODI)
Given the changes in customer behaviour resulting from information and communication (ICT), it is appropriate to seek a deeper and broader understanding of the characteristics of company messages, its links to consumer engagement behaviour on social media (SM), and business performance. In addition, optimizing SM for effective corporate campaigns is an integral part of a company’s daily routine. Despite the fact, that companies collect various data on customer behaviour on SM, traditional analytical methods do not allow for complex processing and forecasting of customer behaviour and improvement of company’s performance (e.g. sales). This can be achieved through Artificial Intelligence (AI) solutions that enable companies to manage the effectiveness of SM campaign results, which can ensure their competitiveness in the marketplace.
Contact: Ineta Žičkutė
—
ArtiMining: The impact of artisanal mining – evidence from Sub-Saharan African countries
The aim of this project is to explore the impact of artisanal mines (legal or illegal) on the economic development of Sub-Saharan African countries from 2000 to 2021. This project proposes to collect exhaustive information for all Sub-Saharan African countries on the opening/closing of artisanal mines with GPS coordinates. I make use of satellite image time series and machine learning tool, namely convolutional neural network, to identify precisely the location of artisanal mines. Equipped with this original dataset, I will address the first order question of the local impact of artisanal mining, at a very large scale. Notably, I will study the local impact of artisanal mines on individual income, health, migration and conflict.
Contact: Mathieu Couttenier
—
AREP: Automatic invoice audtiting
Review and evaluation of possible further developments of automation solutions for invoice audtiting based on machine learning and deep learning methods.
Contact: Simon Hirländer
—
B2B Data Sharing for Industry 4.0 Machine Learning
With the emergence of machine learning (ML) techniques into Industry 4.0 applications, increasing volumes of data are required to train ML applications. This project explores novel business models for business-to-business (B2B) data sharing and designs new methods and tools to govern data sharing – supporting data ecosystems. We 1) explore how mutual benefit of pooling data from multiple organizations may be balanced with their business values, and 2) design technical solutions to support versioning, encryption, differential privacy, licensing, maintaining and collaborating around shared data sets.
Contact: Per Runeson & Christian Kowalkowski
—
CogFinAgent: Integrating human cognitive traits in synthetic intelligent agents to understand market dynamics
Understanding how individuals interact in large-scale social and economic structures, e.g. financial markets, is a key challenge for artificial intelligence systems. On the one hand, we need to grasp how markets shape decision making and on the other, it is crucial to grasp how human cognitive traits impact and structure market dynamics. We aim to characterize these feedbacks using intelligent agent-based models (ABM-AIs) whose properties link individual and macro levels. We will combine ABM-AIs with a computational neuroscience approach to endow agents with realistic learning and key human cognitive traits (impulsivity, confirmation bias, imitation) to understand how they impact market dynamics. We will then turn the tables and use the developed ABM-AI market model platform to structure human experiments, to study how the macro-level impacts individual cognition and patterns of decision making in the financial market. Finally we will then use our ABM-AI platform to analyze a large-scale trader-resolved database to identify the propensity of biases in populations of professional traders.
Contact: Boris Gutkin
—
Collective decisions in Law and Economics: A computational perspective
Courts, parliaments and company boards are just some obvious examples of collective bodies that take decisions after a phase of careful deliberation. Society is driven by group decision-making at all levels. Developing mathematical and computational methods to analyze such decisions and, ultimately, better support them is the long-term vision behind our project. We will be looking at different instances of collective decision-making with a specific focus on decisions that are relevant for the law or the economy, and try to gain insights into their workings by means of mathematical models and computational tools.
Contact: Davide Grossi, Alessio Pacces & Giuseppe Dari-Mattiacci
—
Data Driven Computational Models for Prediction and Simulation of Path Dependencies in Complex Dynamic Labour Market Systems
This project is addressing the need to understand and gain evidence based insight into complex socio-economic and environmental dynamics of Saudi Arabia’s labour market.
Contact: Faiyaz Doctor
—
Decision Support Systems in Digital Business
The research program „Decision Support Systems in Digital Business“ is focused to comprehensive study of complex organizational systems management be it manufacturing, service, social, ecological or virtual. The organizational system inevitably incorporates groups of people working together to achieve common goals. These systems are managed by feedback information, real time and anticipative information, which is provided by decision support systems and other information systems (IS).
Contact: Andreja Pucihar
—
Deep Learning for Credit Risk Assessment
Within the scope of the project deep learning models will be studied and novel deep learning methods developed specifically for the application of credit risk assessment. The goal is to build competences in applications of deep learning models for credit risk assessment for retail and SME customers, and transfer the structured knowledge to the banking industry.
Contact: Zvonko Kostanjčar
—
Deep Reinforcement Learning Algorithms for Risk Management
The goal of this project is to develop a novel class of risk-sensitive reinforcement learning algorithms in dynamic environments with applications in financial risk management. The objectives also include the implementation of state space representation models that extract information from time series data by exploiting latent factors and design of portfolio optimization algorithms based on proposed methods.
Contact: Zvonko Kostanjčar
—
Design of Machine Learning Based Algorithm for Personnel Scheduling (APTS)
Personnel scheduling problem with respect to the workforce demand and rostering has been a subject of research for a long time. Due to the high complexity of the problem, the optimal solution cannot be found in a reasonable amount of time. That is why new methods or their combinations are suggested for automatic scheduling with respect to the known set of constraints. Obviously, employers want employees to perform as many tasks as possible during their working time. On the other hand, they pay attention to the employee’s requests by suggesting flexible working hours, desirable sequence pattern of working days and work time. Optimization with a large set of constraints is not practical if a combination of evolutionary algorithms with the greedy approach is applied. It is planned to design a machine learning based algorithm and implement its prototype during this project.
Contact: Dalia Čalnerytė
—
Digital Asset Management using artificial intelligence – DAMIALabs
Today, the life cycle of media content has greatly accelerated with multi-channel circuits: e-commerce, catalogs, social networks, etc. The joint laboratory (LabCom DAMIALabs) proposed by the CNRS XLIM institute and the company Einden is positioned in this context of DAM solutions, with the central issue of indexing and searching media for large databases of thematic images or associated with a documentary collection.
We propose to design and deploy methods coming from Artificial Intelligence (AI) and data mining, allowing to characterize, organize and index media bases. Methodologically, we propose to rely on approaches based on „raw“ images or on modeling (with a competition) and to exploit machine learning methods by integrating the expert in the loop.
Contact: Philippe Carre
—
Économétrie et Statistique
Le département d’économétrie mène des travaux de recherche théoriques et empiriques en économétrie, statistique et apprentissage machine. Ses membres s’intéressent à de nombreux domaines de l’économie, tels que l’environnement, la santé, la finance, le travail, l’éducation, le développement et les inégalités.
Contact: Emmanuel Flachaire
—
Fintech and Artificial Intelligence in Finance – Towards a Transparent Financial Industry (FinAI)
We want to facilitate interactions and collaborations between different groups of academics and industry working on Fintech and AI in Finance, to provide theoretic expertise to industrial partners, and to establish a large and vibrant interconnected community of excellent scientists across diverse fields. The key objectives are: to improve transparency of AI supported processes by developing a data-driven rating methodology.
Contact: Oleg Deev
—
GREENFIN: Effective green financial policies for the low-carbon transition
By bridging disciplines and using novel data, GREENFIN will lead to a breakthrough in our understanding of the effects of financial policies on the low-carbon transition in non-financial sectors, and thereby lay the foundations for a new interdisciplinary field of financial policy analysis. First, combining theory from technology innovation studies and financial economics, I will derive how technology-inherent characteristics predict the demand for different types of finance. Second, using policy analysis tools and novel machine learning-based methods, I will analyze the effects of green financial policies on the supply of various types of finance. Third, combining these insights I will deliver specific recommendations for designing more effective green financial policies in the EU and beyond. By including financial policies in policy mix analyses, GREENFIN will crucially increase the overall analytical power of public policy analysis, which is all the more important given the ever-growing role of the financial sector in modern economies.
Contact: Bjarne Steffen
—
i-Twin: Semantic Integration Patterns for Data-driven Digital Twins in the Manufacturing Industry
i-Twin investigates integration concepts for data-driven digital twins in the manufacturing industry. The project develops an open source middleware for the integration of operational management systems based on semantic integration patterns. It aims at reducing the integration effort and allowing exchange of master data and operational data in asset management scenarios. The results are validated in a laboratory set-up and in an asset management scenario.
Contact: Christian Borgelt
—
Inequality and Entrepreneurship
This research is at the intersection of organizations and Entrepreneurship. Specifically, the project examines the impact of organizational contexts such as bureaucracy and wage inequality on an employees propensity to venture into entrepreneurship, specifically looking at the careers of knowledge workers from the science and technology labour force. It moves away from conventional research by not only examining entrepreneurial entry but also entrepreneurial outcomes, namely, entrepreneurial performance and entrepreneurial exit, and examines its research through the sociology of entrepreneurship. Methodologically, the project uses quantitaive methods drawing on Swedish labour market provided by Statistics Sweden (SCB). The long terms goal is to apply computational social science methods such as machine learning, Agent Based Modeling and network science into entrepreneurship research.
Contact: Vivek Kumar Sundriyal
—
MIAMI: Machine Learning-based Market Design
First, we propose to develop a new, automated approach to design mechanisms with the help of machine learning (ML). In contrast to prior ML-based automated mechanism design work, we explicitly aim to train the ML algorithm to exploit regularities in the mechanism design space. Second, we propose to study the „design of machine learning-based mechanisms.“ These are mechanisms that use machine learning internally to achieve good efficiency and incentives even when agents have incomplete knowledge about their own preferences. In addition to pushing the scientific boundaries of market design research, this ERC project will also have an immediate impact on practical market design. We will apply our techniques in two different settings: (1) for the design of combinatorial spectrum auctions, a multi-billion dollar domain; and (2) for the design of school choice matching markets, which are used to match millions of students to high school every year.
Contact: Sven Seuken
—
MLEforRisk: Machine Learning and Econometrics for Risk Measurement in Finance
The growing use of artificial intelligence and Machine Learning (ML) by banks and Fintech companies is one of the most significant technological changes in the financial industry over past decades. These new technologies hold great promise for the future of financial services, but also raise new challenges. In this context, the MLEforRisk project aims to provide better understanding of the usefulness of combining econometrics and ML for financial risk measurement. This project aims to provide a rigorous study of the benefits and limitations of these two approaches in the field of risk management, which is the core business of the financial industry. MLEforRisk is a multidisciplinary project in the fields of finance and financial econometrics which brings together junior and senior researchers in management, economics, applied mathematics, and data science.
Contact: Christophe Hurlin
—
Nowcasting of economic activity using real-time big data
The health, economic and social crisis caused by the COVID-19 pandemic has highlighted the need for continuous economic activity monitoring and nowcasting. However, most traditional econometric models rely on historical macroeconomic indicators with relatively significant lags, which diminishes the accuracy of economic forecasts as well as the relevance of the measures targeted at regulation of the economy. Therefore, within the framework of this project, an interactive tool for nowcasting economic activity in real-time, using big data and artificial intelligence methods will be developed.
Contact: Vaida Pilinkienė
—
On intelligence and networks – OCEAN
It appears most timely to develop the theory and practice of a new form of machine learning that targets heterogeneous, massively decentralized networks, involving self-interested agents who expect to receive value (or rewards, incentive) for their participation in data exchanges. OCEAN will develop statistical and algorithmic foundations for systems involving multiple incentive-driven learning and decision making agents, including uncertainty quantification at the agent’s level. OCEAN will study the interaction of learning with market constraints (scarcity, fairness), connecting adaptive microeconomics and market-aware machine learning. OCEAN will shed new light on the value and handling of data in a competitive, potentially antagonistic multi-agent environment, and develop new theories and methods to address these pressing challenges.
Contact: Eric Moulines
—
Risk Management of the Trade Processess Involving Big Data Analytics and Artificial Intelligence
The essence of the project is to develop a model for risk assessment and management of sales processes that will help companies identify and manage risks related to customer reputation, solvency, tax avoidance through artificial intelligence and big data management systems. Research and development of the necessary data collection and evaluation algorithms would also result in creating an IT tool prototype, which will gather data from different sources, different data formats, high speed data into one large database.
Contact: Gerda Žigienė & Robertas Alzbutas
—
Smart Models for Agrifood Local vaLue chain based on Digital technologies for Enabling covid-19 Resilience and Sustainability – SMALLDERS
The overall objective of the project is to carry out research and development activities with the aim of identifying innovative strategies and business models to increase the resilience of small-scale farms in the Mediterranean area, to adequately face disruptive events. The five specific objectives (SOs) of the proposal are explained below: SO-1 – Increasing saleability and perceived value of smallholder products, to be resilient and address any supply chain disruption in the event of a crisis; SO-2 – Increasing smallholder products traceability, quality, safety and perceived value; SO-3 – Helping smallholders to cope with the shortage of workforce due to the COVID-like crisis; SO-4 – Helping smallholders to increase the farm production efficiency; SO-5 – Increasing the Multi-Capital Sustainability of Smallholders processes.
Contact: Francesco Longo
—
The Governance of Economic Activity in the Digital Age
Thus, the overarching research question that constitutes the liaison among the various research interests of TILEC members boils down to the following: Taking into account the growing digitalization of society, exemplified by the increasingly important role that big data and artificial intelligence play for the functioning of markets and other forms of social interaction, how should institutions and structures be designed and what types of incentives can be adopted so as to ensure that public policy objectives are attained?
Contact: Tilburg University
—
Unlocking Frontier Technologies in Firms: Insights from the Past, Challenges for the Future – TECHNOFIRMS
We first plan to leverage the unique richness and granularity of the French information system about firms that is available from French administrations. The recent development of advanced machine learning methods (together with complementary case studies to validate them) has the potential to deal with heterogenous statistical sources and detect technology users at a large scale. This would allow constructing the first population-wide matched firm-worker dataset allowing to address our research question.
We will then investigate the economic mechanisms and societal infrastructures that lead to the adoption of new technologies and determine their impact via two main axes of investigation. The first axis will investigate the interplay between firms’ environments and their technology adoption decision. Our second axis of investigation will focus on the role played by labour, and more precisely by the way it is organized, in determining whether and which technologies are adopted, what are the productivity gains and how they are split between stakeholders.
Contact: Claire Lelarge
—
VADORE: Data Valuation for Job Search
AI to improve the job market: new functionalities available for job seekers and job providers. The central idea of the project is to mobilize all available information to improve the matching of job seekers and job vacancies.
The project is based on the mobilization of the considerable body of information on job seekers and firms, some of which (notably textual data) is still untapped. This information will be used to develop, rigorously evaluate and compare two different functionalities, of different technical and economic inspiration. The first functionality consists on issuing formalized recommendations to both job seekers (about the companies to apply to) and companies (about the job seekers to invite for recruitment interviews) using previous work. The second functionality consists on making available to job seekers and companies an interactive map of employment, allowing them to view in an aggregated way job offers and job applications in a geographical area and responding to a given request.
Contact: Michèle Sebag, Marco Cuturi, Bruno Crepon, Christophe Gaillac & Philippe Caillou
Film Studies
AI and Story Telling
An exciting fusion of creative writing and artificial intelligence to help writers create new forms of dynamic, interactive stories. It specifically aimed to understand what the impact of artificially-intelligent memory is on storytelling and narrative structure, and how to transfer the resulting research into industry.
Contact: James Smithies
—
Artificial Vision & Intelligence for Visual Effects – ALICIA-Vision
The photorealistic combination of computer-generated images and film footage images of a same scene is a central objective in the creation of visual effects for the cultural and creative industries. The constant advances in numeric algorithms and computer computing power have made artificial vision (also known as computer vision) a mature discipline so that a large number of dedicated applications are now being used in these industries. While these new technologies have profoundly changed workflows, many tasks remain manual. Very recently, thanks to the increasing prevalence of very large datasets and the ability of computers to process them, advances in artificial intelligence (AI) for machine vision, and in particular machine learning, have created a genuine scientific revolution. These advances make it possible to envisage a new qualitative leap in the performance of tasks required by visual effects, by “learning” computers how to perform these tasks using examples, in order to complete or replace certain „classic” artificial vision algorithms, which today limit the implementation of fully automated processing.
Contact: Pierre Gurdjos
—
deep-doLCE: A New Machine Learning Approach for the Color Reconstruction of Digitized Lenticular Film
The deep-doLCE project explores a more advanced and robust method, using an already available big dataset of digitized lenticular films to train a new deep learning software. The aim is to create an easy-to-use software that revives awareness of the lenticular color processes thus making these precious historical color movies available again to a public and securing them for posterity.
Contact: Giorgio Trumpy
—
Deep learning in film analysis
In VIAN kommen zusätzlich zu manuellen Methoden Deep Learning Tools zum Einsatz, welche unter anderem eine Figur/Grund-Trennung vornimmt oder Figuren und Gender automatisch erkennen kann. Nach und nach implementieren wir zudem automatische Analyse von Bildkompositionen, visueller Komplexität, Farbverteilungen, Mustern und Texturen. Die Filme werden automatisch segmentiert, Screenshots erstellt und gemanagt.
Contact: Barbara Flückiger
—
The face in film and media art
The project explores the reflection of biometric facial recognition and algorithmically controlled security dispositions in contemporary photography, film and media art.
Contact: Winfried Pauleit
Literary Studies + Linguistics
Affective Language Production: Content selection, message formulation and computational modelling
In this project we study how speaker’s emotional state influences the language that they produce, looking both at the early stages (content selection) and later stages of language production (message formulation). In addition, we develop a computational model that generates emotionally charged texts, paving the way for targeted news-reporting.
Contact: Tilburg University
—
Artificial Intelligence for creative language use
Recent progress in Natural Language Processing (NLP) has resulted in reliable pattern matching techniques (mostly based on deep neural networks) for many NLP tasks (text to speech, speech to text, text generation, text translation, multimodality, text analysis, …). The creative use of language (e.g. in advertising slogans, song texts, humor, irony, metaphor, …) has remained out of reach of current approaches. We will investigate how the improved stated of the art in ‚literal‘ language processing can push the design of creative language processing systems. Valorisation roadmap: The research address two types of users and applications: (i) professional writers who will be able to use tools to generate ideas and concepts (puns, jokes, titles, short texts with metaphors) and (ii) language enthusiasts who will be provided with tools that can boost their output by producing examples and ideas.
Contact: Walter Daelemans
—
BOSS 1.0: Biblical Online Synopsis Salzburg 1.0
This project is part of an international effort to develop an open-access online tool (BOS) that allows ancient versions of biblical scriptures to be compared with each other at the textual, syntactical and semantic levels. Until now, synopses, whose information content is limited to a double-page spread, have only been produced in manuscript form or as printed editions. BOS will not only become the first freely online accessible hub for all digitised Bible texts, but will itself offer analysis possibilities based on the latest approaches from the field of machine learning. The project will initially create a manual synopsis for the Book of Joshua. The synopsis forms the basis for the cooperation partner from computer science. The idea is to automate parts of the manual analysis with the help of machine learning and statistical methods and then verify them using the manually generated results. Such approaches have already been tested in the evaluation of philosophical texts, but have never been systematically applied to biblical texts. The difficulty lies in the fact that the texts are in different ancient languages and there are hundreds of years between their creation. This requires a systematic evaluation of existing techniques and, in all likelihood, an extension to include novel approaches.
Contact: Database Research Group Salzburg & Kristin De Troyer
—
CODIM: Compositionality and Discourse Markers
The CODIM project focuses on the two main linguistic resources for organizing monologues or conversations in human languages : D(iscourse) M(arkers) (therefore/donc, well/ben,bon etc. in English/French) and prosody (in particular intonation). It will evaluate their status with respect to two major views on communication: compositionality (the possibility of combining meaningful expressions into more complex meaningful expressions) and pattern or construction-based approaches (the idea that language users exploit partly ‘frozen’ strings of words). We will compare the semantic and prosodic properties of simple and complex French DM (e.g. ah + bon) found in corpora for written and spoken French, using a variety of complementary approaches for DM identification (category-driven text mining), clustering (statistics and Machine Learning) and research in prosody (ToBI representation, speech analysis/synthesis). This will foster or reinforce strong collaborations between linguists and computer scientists.
Contact: Mathilde Dargnat
—
CorVis: Translating sign language with computer vision
The CorVis project aims to develop cutting-edge sign language translation techniques, based on recent advances in artificial intelligence, in particular in the fields of computer vision and machine translation. Sign language analysis from video data is understudied in computer vision despite its great impact on the society, among which is enhancing hearing-deaf communication. This project, therefore, will make a step towards translating the video signal into spoken language, by learning data-driven representations suitable for the task, through deep learning. There will be two inter-related directions focusing on: (1) the visual input representation, i.e., how to embed a continuous video sequence to capture the signing content, (2) the model design for outputting text given video representations, i.e., how to find a mapping between the sign and spoken languages.
Contact: Gul Varol
—
DIgital investigation of Phonetic VARiation: large-scale multilingual modeling of lenition and fortition – DIPVAR
We propose to use speech technologies and artificial intelligence methods for the multilingual study of phonetic variation. Specifically, we will study consonant lenition and fortition, which are both sound changes and patterns of synchronic variation. Our hypothesis is that variation is multifactorial and goes beyond classical linguistic measures and that by focusing on variation at the present time we can understand diachronic mechanisms and make assumptions about future changes. We propose to merge linguistic approaches and numerical methods, including techniques based on speech recognition and machine learning, in a project dedicated to language exploration that combines acoustic and prosodic descriptions and typological perspective.
Contact: Ioana Vasilescu
—
ExPLAIN
The project will uncover missing explanatory links in argumentative texts, fill in automatically acquired knowledge that makes the structure of the argument explicit and establish and verify the knowledge-enhanced argumentation structure with a combination of formal reasoning and machine learning.
Contact: Anette Frank
—
Intergramm
This empirical research project investigates the language elaboration of Middle Low German from the 13th century to the written language shift in the 16th/17th century. At this time, Middle Low German lost its dominant position as a supraregional written language to Early New High German. This study makes an important contribution to the reconstruction of grammatical developments in written Middle Low German as historical written language, which are hitherto examined only to some extent.
Contact: Doris Tophinke
—
KEDiff: Cooperative indexing of diffuse knowledge. A literary-informatics model experiment for the processing of complex text corpora using the example of the „Oberdeutsche allgemeine Litteraturzeitung“ (1788-1811)
In 1788, the „Oberdeutsche Allgemeine Litteraturzeitung“ (OALZ), a review journal, was founded in Salzburg. From the beginning, it was dedicated to the „promotion of a true Enlightenment“ and was published until 1811. Also because it took into account the Catholic book production that had been passed over by the other journals, it quickly developed into one of the most important media of self-understanding for the South German Enlightenment. However, due to its textual volume and broad thematic distribution, its content has not yet been adequately explored.
An integral analysis of individual volumes, or at best of the entire journal, can be expected to yield considerable insights for Enlightenment research. At the same time, a methodological model is to be developed with the help of which other similarly complex text corpora can be researched in the future – in addition to other historical text collections, also digital text formats such as blogs or postings on social media platforms.
In order to avoid the stereotypical criticism of a feared or actual juxtaposition of ‚intensive‘ historical reflection and ‚extensive‘ statistical analysis or machine processing, the project is decidedly designed as a cooperation between German studies and computer science, with the professional expertise and strengths of both disciplines being communicated in each project phase.
Contact: Christian Borgelt
—
Machine Translation and Literary Texts: A Network of Possibilities
For years literary texts have been off limits for machine-translation editing. This is beginning to change, but research on this subject tends to focus on productivity. We know little of what technology does to literary texts. This project will examine how machine-translation editing and the way the text is presented to translators on screen affect literary translations.
Contact: Lucas Nunes Vieira
—
Mardy
This interdisciplinary collaboration project involving Computational Linguistics, Machine Learning and Political Science has the aim of developing new computational models and methods for analyzing argumentation in political discourse – specifically capturing the dynamics of discursive exchanges on controversial issues over time. The goal is to develop tools to support analysis of the possible impact of arguments advanced by different political actors.
Contact: Jonas Kuhn
—
Natural Language Inference and Inference in Natural Language
Humans draw inferences from available information all the time, as an essential part of our decision-making process and in a manner that crucially informs and sometimes determines action. This human ability has been carefully studied with modern methodologies within the social sciences (linguistics, philosophy, cognitive psychology) over the past 70 or so years. More recently, research in AI has made it a priority to train natural language processing models to make inferences over naturalistic text in a way similar to humans.
However, current work from within AI on inferences drawn by language models is making insufficient contact with the empirical discoveries and theoretical advances of the domains of the social sciences that specialize in describing and explaining the relevant human faculties. This project proposes to advance our convergence between natural-language inference in AI and crucially related work in linguistics, philosophy, and cognitive psychology chiefly by investigating two points of contact: pragmatics and the notion of alternative possibilities.
Contact: Salvador Mascarenhas
—
OTELO: OnTologies pour l’Enrichissement de l’analyse Linguistique de l’Oral
OTELO proposes a multi-level analysis of spoken language from large oral corpora, segmented and annotated automatically. Segmented into phones and words, these data will then be enriched with knowledge about the grammatical status of words, their syntactic and semantic relationships in context. The expected results concern: the role of phonetic information in the disambiguation of contextual homophonies involving entities; the impact of „high-level“ linguistic knowledge (grammatical, syntactic, semantic) in the diffusion of patterns of phonetic variation within a language’s lexicon.
Contact: Fabian Suchanek & Ioana Vasilescu
—
The beginnings of modern poetry – Modeling literary history with text similarities
While today only a small group of poets and poems is seen as ‘modern’, the contemporaries applied this attribute to much more texts. Does literary history ignore the modern trends in those other poems or did the contemporaries perceive change and innovation where there was none? To answer this question, the development of new methods will concentrate on semantic text similarity and sentiment or more exact emotion analysis. Determining which approach to word embeddings is preferable for our use cases and how they can be used to represent short texts focusing on dimensions like general semantics is one focus. The other is the development of an historical sentiment lexicon including emotions without anachronism.
Contact: Simone Winko & Fotis Jannidis